Demand forecasting is the process of predicting how much demand your products will have over a specific period, based on historical and real-time data. It helps make the right procurement and supply decisions for the business and its customers.
As a retailer, demand forecasting must be routine for you, whether you sell 1,000 SKUs or 10 million. In fact, the higher the number of products you sell, online or offline, the more important it is that you forecast the demand for your products accurately for the upcoming months.
Why Is Demand Forecasting Essential in Retail?
Demand forecasting is essential for almost every activity from production or procurement planning to sales and marketing to assortment planning.
It is a critical BAU activity for several reasons, such as:
- To balance product availability with minimal stock risk—cut down inventory issues and wastage at the same time
- To ensure you are able to procure the right amount of inventory required to meet customer requirements in the near future: both online and offline
- For optimal inventory planning and management and to avoid out-of-stock as well as excess or old stock scenarios
- To understand which products are needed in approximately what quantity at each store
- To know how much inventory your warehouses should store to meet consumer needs on your digital channels
- For capacity management—ensuring that production/supply and in-store efficiency is aligned with the projected demand
- To make supply chain management more efficient by helping you decide the inventory required for each product category and whether more or fewer suppliers would be needed at a time
- To be able to create, produce, procure, or design new products to meet customer needs better
- For planning production requirements and logistics, if you are a D2C brand that manufactures your own products
- To be able to do assortment planning the right way so that products not being sold during a particular period do not take up key shelf spaces
- To optimize cross-sell and upsell strategies around alternative and similar products
- For optimization of product promotion campaigns and advertising spends, i.e. knowing which products to promote through discounts and offers and which not to
- To reduce operational costs and increase profitability
What Are the Traditional Demand Forecasting Methods?
Once upon a time, demand forecasting was siloed to individual stores, and having one individual dedicated to tracking product movements and predicting requirements was enough.
But in the past decade, with different sales channels—multiple stores (many a times in different countries), websites, and apps—it is important to have an omnichannel outlook to forecasting.
The scale of omnichannel means that the amount of data—related to both product movement and customer behavior—is massive, which is beyond the scope of a few individuals and their spreadsheets.
Traditional demand forecasting methods consist of two key areas:
-
Quantitative methods, which employ mathematical and statistical models to understand the trend and results. These include models such as Percentage Over Last Year, Moving Average, Linear Approximation, Exponential Smoothing, Lifecycle Modeling, Time-series Modeling, Regression Analysis, and Econometric Modeling.
-
Qualitative methods, which are subjective and sociological methods of collecting information and applying ideas generated from them to the problem at hand. These include Market Research, Historical Analogy, Expert Opinions, Delphi Method, Panel Consensus, and Focus Groups.
Why Use Machine Learning for Demand Forecasting Instead of Traditional Methods
As is obvious, most traditional demand forecasting methods are manual in nature, relying on collecting information and analyzing them using spreadsheet formulae.
But when your retail data points run into millions and the variables that determine the demand for a product run into dozens, manual forecasting is simply time-consuming and prone to human error.
In addition, it is impossible to consolidate all data points and all kinds of different analytical models into a single spreadsheet or chart for a 360-degree view—inevitably, some factors get left out and siloed interpretations follow.
You might find one statistical model telling you that you need to stock up on baking essentials because it’s Thanksgiving. Another study tells you baking is falling out of fashion because people are working more and have less time for personal activities. And then, a third unknown factor of sudden bad weather drops out of nowhere. So, should you stock up on baking essentials or not, and how much?
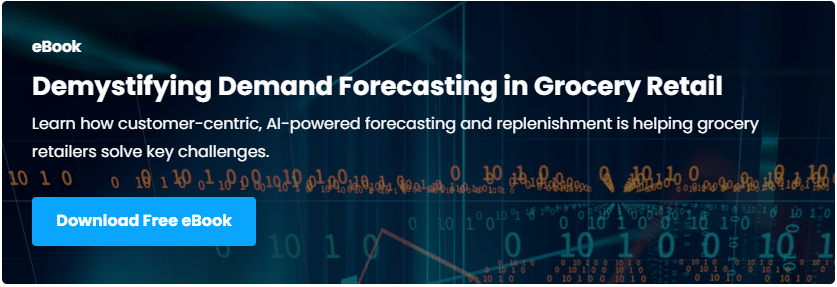
9 Ways Retailers Can Benefit from Machine Learning in Demand Forecasting
Today’s retailers must have accurate demand forecasts in order to optimize every part of the chain of activities required to meet the day-to-day appetite for their products. The better forecasts you build, the more efficient each of your procurement, sales, and marketing processes will be.
And nothing can give you better data accuracy than machine learning-based software.
McKinsey notes that using ML and AI in demand forecasting and supply chain management can reduce errors by up to 50% and reduce lost sales and product unavailability situations by 65%. This can lower warehousing costs by up to 10% and administration costs by up to 40%.
These benefits are surely too good to pass up.
For starters, AI algorithms use a combination of the best of mathematical, statistical, and data science models. An ML-based forecasting software doesn’t simply apply past patterns within a business to predict future requirements; it evaluates each factor likely to impact demand in real time, and automatically gives you a constantly updated picture of sales, demand, and inventory.
Machine learning can process millions of data points in minutes, draw trends and insights across different dynamic conditions, and show you how each variable affects another and thereby the overall demand. It can find non-linear connections between variables, which are crucial for the best forecasting models.
Plus, these algorithms constantly learn from the data the software ingests. It is already trained on several forecasting models and historical data, and further training with real-time data strengthens its accuracy. This helps you automate the whole process and cut down on the human hours required for the task.
All this makes predicting demand through machine learning accurate, fast, and scalable, which, in turn, ensures efficiency in the entire supply-to-sales chain.
To summarize, using machine learning for demand forecasting can benefit you in the following nine ways:
- Process more data points than a human can
- Process data from more sources
- Process the data quickly
- Identify hidden trends and insights from the data
- Identify relationships between the variables that impact demand
- Generate accurate forecasts by factoring in several variables
- Automate and update the forecast in real time
- Make the forecasting system robust, scalable, and adaptable
- Save time, money, and resources by making every step of the supply-to-sales chain effective and efficient
Related Blogs
7 Demand Forecasting Challenges Machine Learning Can Solve
Let’s see how ML algorithms can help retailers deal with the many challenges that demand forecasting inherently presents.
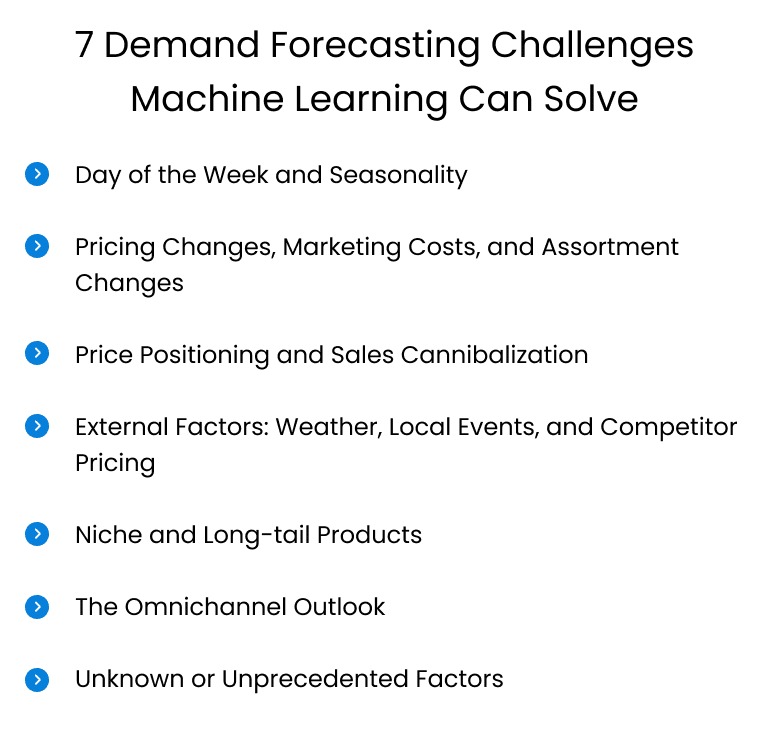
-
Day of the Week and Seasonality
Weekday versus weekend sales and higher or lower sales of certain items in specific seasons are things every retailer contends with every day. A simple time-series modeling might help you determine these patterns easily.However, machine learning’s accuracy comes from the fact that these clever algorithms find how these variables and demand are related. It also factors in other variables, such as offers, promotions, and weather, ensuring accuracy and giving you a 360-degree view of where your product’s demand would stand in the next few days or weeks or months.
-
Pricing Changes, Marketing Costs, and Assortment Changes
Offers, promotions, discounts, in-store display changes, and investment in online and offline marketing campaigns, can affect how the appetite for the product shapes up. It’s difficult to predict the impact each of these factors can have on demand, without some really complicated number crunching.Machine learning can do the heavy lifting for you and accurately predict how a product’s price change can affect its demand. This helps not only in forecasting but also in understanding promotion forecasting, markdown optimization, assortment planning, and marketing campaign management.
-
Price Positioning and Sales Cannibalization
The price difference of a product compared to other products in the same category also affects demand. For example, the highest priced product in the category may end up not getting sold at all.Similarly, promotions and discounts of one product in a category could bring down the demand for other products in that category.
Keeping track of these phenomena for each category of products you sell can be back-breaking. However, ML algorithms learn from each piece of data, and therefore can give you a comprehensive view of factors impacting the demand of each product not only within itself, but also in relation to other products in the category.
-
External Factors: Weather, Local Events, and Competitor Pricing
Demand is sometimes heavily affected by external factors, such as weather, local crowd-pulling events, and pricing changes and promotions by competitors. Without machine learning-based automation, these things are almost impossible to be factored into demand forecasting.ML algorithms can quickly and accurately map the relationships between weather and sales at a localized level, giving a granular outlook on the market for your products. They not only detect which product would be in demand during a weather pattern, but also tell you what product would not be needed.
The same goes for understanding how a big concert or game near the store or in a region can affect demand for certain products, or how promotions being run by competitors or new stores/online outlets can change footfall/traffic to your channels. You only need to feed the right data into the ML-based tool you use.
-
Niche and Long-tail Products
Many niche products have negligent sales data because barely a few units are sold each month. This leads to a scarcity of data on the item and unpredictable variations in demand patterns for the product.Add external factors and cross-channel variables, and the output can actually become unreliable. However, robust and self-learning algorithms can cut out the noise, avoid overfitting, and arrive at close-to-accurate results for niche products as well.
-
The Omnichannel Outlook
Several forecasting challenges are often unique for in-store and online channels. Even within each channel and each store, there are variations depending on location, logistics, shelf space, personnel availability, etc.Machine learning makes it possible for retailers to not only get an overview across stores and channels, but also look at the requirements of each individual store and channel.
Because of this, it can suggest internal stock movements easily. For example, say your Pittsford store has an excess stock of peanut butter and your Rochester store is running out of it. Your ML tool can make this information more visible. So, instead of urgently procuring fresh stock for Rochester, you can move some of the stock from Pittsford and meet the requirement quickly.
The same thing can be done cross-channel; the algorithms can suggest when excess in-store stock can be moved to the online inventory and vice versa.
-
Unknown or Unprecedented Factors
Machine learning algorithms also allow you to factor in unknown factors impacting demand. In 2020, for example, the pandemic was a sudden and unprecedented factor that changed consumer needs overnight. An E2open study found that amid the pandemic, real-time data and AI-powered analysis reduced forecast errors by over 33%.ML software can add a tentative input in the forecasting model, making it ready to update the numbers within minutes of adding in a new datapoint. Retailers can also do what-if simulations to analyze how changes in variables can affect demand, so as to be prepared for unknown factors and reduce forecasting errors.
Unknown or unprecedented data can be best handled by a machine learning tool if it has real-time data processing capabilities. Inputs such as search trends, social media actions and hashtags, global and local news, and other non-linear and unstructured data help machine learning algorithms increase the accuracy and value of their output.
Time to Add Machine Learning to Your Demand Forecasting Process
Now that you know the immense benefits machine learning can bring to how you forecast demand, time to look at different ML-based software and get one for your business. Algonomy’s Forecast Right is one such AI-driven forecasting solution that is also easy to use.
Sounds interesting? Learn more or Get a demo to decide for yourself.