The Ultimate Guide to Demand Forecasting in Grocery Retail (2024)
This guide is a tool to help retailers build a foundation of modern demand forecasting. Feel free to navigate through it at your own pace using the table of contents.
Table of contents
- What Is Demand Forecasting?
- Global Grocery Retail – Trends and Stats
- Why Accurate Demand Forecasting Matters More Than Ever in Grocery Retail
- Why Is Demand Forecasting Important in Grocery Retail?
- How Does Demand Forecasting Empower Key Retail Activities?
- Demand Sensing vs. Demand Forecasting: What’s the Difference?
- Demand Forecasting in Grocery Retail – Why Most Frameworks Fail to Produce Desired Results?
- Three Critical Capabilities for an Effective Demand Forecasting Framework
- From Static Models to Intelligent Insights: How Do AI & ML Transform Grocery Retail Demand Forecasting
- Key Advantages of AI-powered Demand Forecasting
- Key Considerations for Choosing the Right Demand Forecasting Solution
- Retail Demand Forecasting With Algonomy – How Can We Help?
Global grocery retail is teeming with disruptions – intense competition leading to shrinkage in retail footprint, nuanced customers transforming online grocery retail from niche to mainstream segment, and brand hopping for diverse reasons ranging from quick and free deliveries to organic produce, subscriptions, and bundle offerings – keeping a track of variables to optimize retail planning strategies is getting out of hand.
While the global food and grocery retail market is expected to hit the USD 12.3 Trillion mark in 2024, there are more than 60,000 supermarkets and grocery store businesses in the US alone. Further, McKinsey reports that by 2030, online channels can account for 30% of the food-at-home market.
This necessitates the need for intelligent demand forecasting and data-driven retail planning. How is intelligent forecasting done and how does it differ from traditional techniques? What makes demand forecasting a powerful tool to navigate the challenging retail landscape, and how can retailers leverage AI-powered automation to continuously plan and execute for disruptions?
Here is a complete guide to demand forecasting in grocery retail that answers all these and many other questions.
What Is Demand Forecasting in Grocery Retail?
Demand forecasting is the process of making future estimates of customer demand over a specific period at a given price point, in a specific time frame and location.
Traditionally, demand forecasting considers historical data and static analysis of different information, such as sales figures, and manually-defined adjustments to seasonal fluctuations to produce the most accurate predictions.
In grocery retail, future customer demands are predicted for specific products (or SKUs) for various store locations to ensure that the wastage is minimal and availability is high, without
However, the modern grocery retail sector requires a granular and real-time approach — monthly, weekly, or daily forecasts to support various category management processes and supply chain decisions. Granular and adaptive forecasts are critical, given the wide spectrum of products varying from fresh to ambient products.
Global Grocery Retail - Trends and Stats
- Grocery retailers today face unprecedented top and bottom-line pressure due to an irrevocably changed grocery environment.
- Globally, grocers lose about $1 trillion in lost sales due to out-of-stock (OOS) in a year.
- Additionally, $750 billion worth of food products are wasted every year.
- Moreover, McKinsey’s research suggests that consumers are challenging brand loyalty by changing brands frequently. 36% of consumers are trying a new brand while 25% have moved to private labels.
Why Accurate Demand Forecasting Matters More Than Ever in Grocery Retail
Grocery retail is undergoing a paradigm shift, and accurate forecasting has far-reaching consequences across category management, customer satisfaction, and supply chain management.
Here are a few noteworthy statistics to emphasize the increasing importance of accurate demand forecasting:
- Research suggests that loss of sales has increased from 5-10% historically to 15% post-pandemic
- Loss due to food wastage is recorded at 30% due to retail store inefficiencies and agricultural waste. The World Bank estimates per person per day food wastage to reach 2 kg, by 2025.
- The cost of holding excess inventory in grocery retail has ballooned up to 30%, reducing the profit margins significantly
- A retailer risks losing up to 10-30% of its revenue if it fails to cater to the omnichannel demand
Why Is Demand Forecasting Important in Grocery Retail?
Disruptions Galore
As the omnichannel grocery spend inches closer to the projected 18% annual rate, the entry of grocery retail category disruptors, like Beyond Meat, Caulipower, HaloTop, etc. are pushing the well-established enterprises to amass more floor space and deeper penetration.
Add to the mix, the nuanced purchase patterns of the consumers – subscription meal boxes, small-business organic foods, and calorie-counted meal portions – and the assortment matrix is looking nothing short of Pandora’s Box.
Market With Evolving Complexities
The enduring nature of the grocery retail challenges is an indication that grocery retailers need to catch up with evolving customer preferences and strengthen their supply chain to ensure that shelves are properly stocked – in stores and online.
It has become increasingly important to get demand forecasting right down to a T. Gaps in On-Shelf Availability (OSA), both online and in-store, can be catastrophic and result in lost volume, lost baskets, and lost faith among shoppers.
Strategic Retail Organization
Demand forecasting is also a very strategic piece of the puzzle in a retail organization. It has a direct effect on the financial health of a retail organization – profit margin, cash flow, working capital, and operational expenses are some of the key business metrics that it affects.
Moreover, it is one of the key enablers of growth and market expansion, which is a business imperative for established players, as mentioned above. Accurate forecasting prevents stockouts and overstocking, and frees up cash flow, allowing for investments in other areas like marketing initiatives or store expansions.
How Does Demand Forecasting Empower Key Retail Activities?
Here are some of the key processes that rely on accurate demand forecasting:
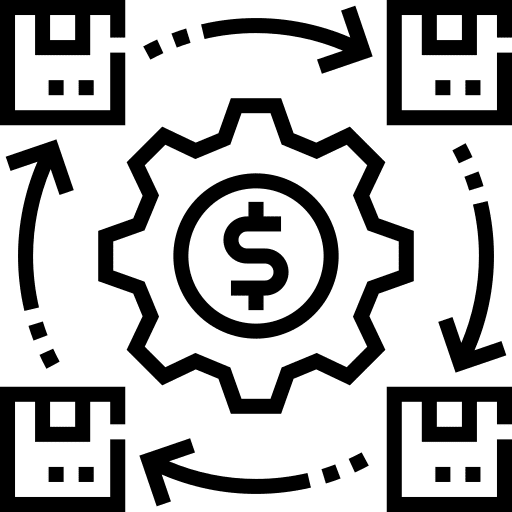
Supply Chain Optimization
Demand forecasting forms the backbone of supply chain decisions such as location, production, inventory, and transportation. By accurate forecasting, supply chain planners can optimize their supply chain to reduce cost, and bring in efficiency and responsiveness.
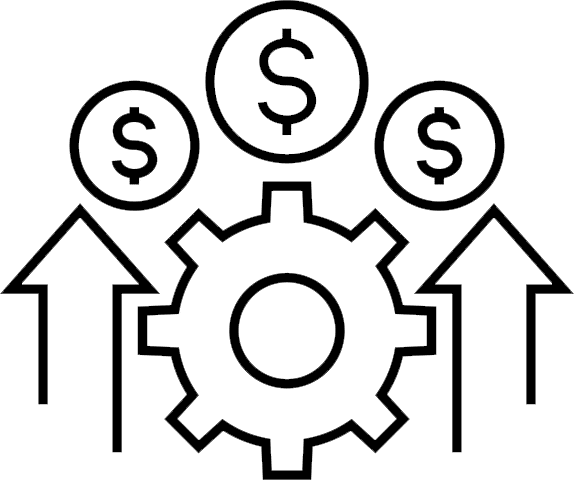
Cost Efficiency and Financial Planning
A strategic emphasis on demand forecasting goes a long way in increasing your cost efficiency and making your business profitable. By planning for trends, peak intervals, and seasonal demands, and accounting for supply-side risks, grocers can optimize working capital and maximize cash flow.
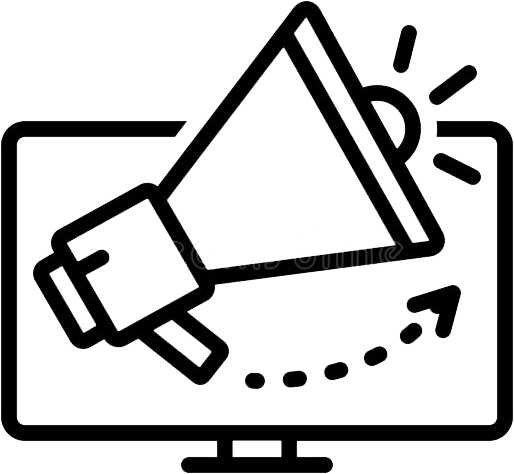
Implement Relevant Marketing Campaigns
With a clear understanding of customer demand, you can make your marketing efforts more targeted, cost-effective, and tailored to your demand curve. Proactive marketing interventions can help you boost sales in lean periods and achieve greater marketing ROI throughout the year.
For example, in the case of reduced sales prediction, running appealing promotional offers and discounts for a limited period will increase the purchase, to balance out the potential loss.
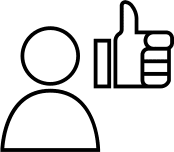
Improved Customer Experience
Customer experience is the key to every business, especially post-pandemic. Consumers value price and convenience over anything else.
Research suggests that 75% of consumers are modifying their purchase behavior to accommodate economic changes, store closings, and evolving priorities.
By understanding customer trends and anticipating customer needs, category managers can stock the products that customers are most likely to want, when and where they want them. This prevents out-of-stock and the customer dissatisfaction that comes along.
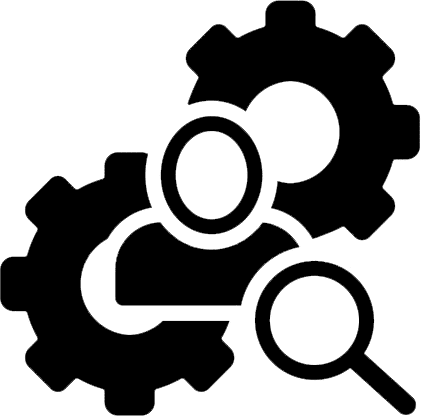
Addressing Staffing Issues
Staffing issues are visible during both peak season and a dip in demand. While a lack of staff can result in customers waiting to get a service, the availability of more staff can be overwhelming. Therefore, predicting retail demand will allow managers to schedule staff shifts accordingly for an elevated customer experience.
Demand Sensing vs. Demand Forecasting: What’s the Difference?
Category managers and supply chain managers would ideally like 100% accuracy in demand forecasting. But the problem is that it does not exist yet due to the vagaries in the business. Digital technologies such as artificial intelligence and machine learning can elevate demand forecasting accuracy up to a certain limit.
Demand forecasting works really well for mid to long-term planning. However, it needs to be complemented with effective short-term planning. This is where demand sensing comes into play.
Demand sensing is a more short-term focused forecasting approach that predicts customer demand on a daily and weekly basis. It leverages ML/AI to factor in a wide range of demand predictors that factor in real-world events that affect customer behavior.
With the help of demand-sensing capabilities, grocers can now more effectively determine where, when, and what products consumers will buy on an ongoing basis. It helps build supply chain resilience, improve inventory management, and better estimate demand.
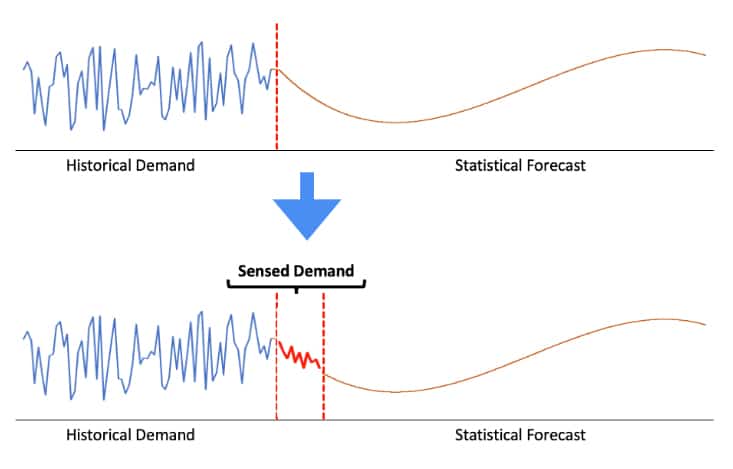
Source: LinkedIn
Demand Forecasting in Grocery Retail - Why Most Frameworks Fail to Produce Desired Results?
One-Size-Fits-All Approach
Traditional forecasting methods use predefined rules and are unable to capture the diversity of the complex product matrix. Every product category and distribution channel has unique requirements and needs a tailored approach.
Static One-Time Forecasts
Traditional forecasts are unable to account for internal factors dictating the aisle. For example, the forecasts do not consider the markdowns, promotional offers, and short-term cannibalization occurring due to the unavailability of competing products.
Reliance on Manual Elements
In the case of sparse data, noisy data, and shorter product life cycles, the traditional forecasting accuracy relies on manual intervention, making it prone to error.
Lack of a Recommendation Engine
Demand forecasting is a highly intensive process with a series of decisions that determine forecasting accuracy. Most of the current tools do a great job of analyzing and visualizing data. However, they miss out on enabling the category managers and supply chain planners to make the right decisions, such as what predictors to use for what category, what model to use given the data, what data to exclude for analysis, etc.
eBook
Three Critical Capabilities for an Effective Demand Forecasting Framework
Every retailer looks for 100% forecast accuracy – the ideal case scenario. However, on average, 70% accuracy is generally considered acceptable.
But then again, focusing solely on accuracy as a measure of forecasting effectiveness has its limitations:
- Absolute Error: Accuracy simply tells you the overall size of the forecast error, without considering if it’s an overestimate or underestimate.
- Category Objectives: Not all forecast errors have the same impact. For example, overestimating sales of shelf-stable (ambient) products is less critical than overestimating fresh produce, which can lead to spoilage and wasted resources.
Hence, we recommend three critical capabilities for creating an effective and reliable demand forecasting framework.
Data Capturing and Enrichment
The demand forecasting framework must capture the myriad forms of data generated across the retail organization apart from historical sales data such as discounts, inventory, and promotions as well as important external factors such as weather and macroeconomic indicators. The framework also needs to be robust enough to tackle modern data challenges such as sparse and noisy data.
Tailored Forecasting
The forecasting framework needs to seamlessly tailor forecasts to channel, store, and category nuances.
For example, the demand for fresh and seasonal products in a particular store would be different from the demand for an ambient product in the online channel. Identifying patterns in each of these combinations and tailoring forecasts to it can go a long way in optimizing stock and winning customers.
Agile Forecasting or Demand Sensing
Forecasting should instill agility in category management. Predominantly, customers react to what is happening on the shelf. If your category management team can react to them preemptively, then that will be a huge win for your retail organization.
For example, effects like cannibalization, out-of-stock, and halo effect can be simulated way in advance, avoiding last-minute panic to stock shelves. The forecasting framework needs to be the go-to tool for category managers to be able to do all of the above-mentioned tasks in a few clicks.
From Static Models to Intelligent Insights: How Do AI & ML Transform Grocery Retail Demand Forecasting
Demand forecasting is a statistical process where a planner makes a series of decisions or forecast plans. Based on them, key decisions are taken across different segments, such as category management and supply chain management.
Traditionally, this is done via static ERP solutions and analytical tools, and owing to the less diverse nature of the retail sector, the forecasts used to be reasonably accurate with a tolerable error. However, in recent years, grocery retail witnessed a seismic shift globally, partly accelerated by the pandemic, and traditional forecasting errors have shot through the roofs.
The inability of traditional static forecasting frameworks to adjust to dynamic market changes and consumer preferences affects the overall effectiveness of the forecasts. Also, category managers need to factor in the right indicators of demand and the right models for each channel, store, and category combination to achieve accurate forecasting, which is not possible in a manually-managed forecast model.
This is where AI and ML come into the picture.
With its inherent abilities to quickly process huge unstructured data sets, identify hidden trends and patterns, and generate predictions for multiple custom disruption constraints, AI and ML-powered forecasting frameworks do a commendable job. They surpass the abilities of manual and static modeling and help retailers unlock accurate forecasting for 90% of the SKUs.
Treating Data Challenges
Your forecast is only as good as your data. While retailers have a sea of data, they often find themselves struggling with noisy and sparse data sets. They either fail to spot these challenges, in which case their forecasting is jeopardized, or depend on external teams to get the data cleaned which is a time-consuming process.
AI can readily treat noisy data coupled with Machine Learning techniques, and identify outliers and invalid data. For sparse data sets, techniques such as attribute-based forecasting can bridge the gap and provide accurate demand curves for new products. All of this can be achieved without the help of any external team.
Enriching Data with Real Predictors of Demand
Traditional frameworks use just historical sales data to create forecasts. This approach, however, has a major flaw – it assumes that future demand patterns will be more or less similar to past demand. It completely ignores the causality aspect.
For example, let us consider weather as a predictor of sales. For categories such as cold beverages and desserts, there is a strong causal relationship between temperature and sales. So if during the forecasting period, the temperature is higher on average compared to last year, then the classical forecasting framework will fail drastically.
The factors that impact demand range from external factors such as weather, holidays, macroeconomic factors, etc. to internal factors such as pricing, inventory, etc.
Incorporating this wide range of information in your forecast is a huge challenge because of data complexity. On the other hand, Machine Learning algorithms can factor in a wide range of predictors and relationships that impact demand on a daily basis into your retail forecasts with just a few clicks.
Selecting Category, Channel, Store-specific Predictors
Demand planners often face the dilemma of choosing the right predictors for each category/product. Oftentimes this decision is made on the basis of past understanding of how the category behaves or even worse with a one-size-fits-all approach.
For example, while demand for meat products goes up sharply during weekends and holidays, demand for chilled beverages is highly correlated with weather. Using the same approach for both categories may prove disastrous, given the stark difference in the predictors for the categories.
AI/ML can recommend what predictors are significant indicators of demand for a channel, store, and category combination. This goes a long way in improving the overall forecasting accuracy.
Finding The Best-Fit Model To Meet Your Category Objectives
Each channel, store, and category behaves differently. There is no “silver bullet” model that can give you the best forecast.
For example, for a fresh product in a small remote store, the selection of the forecasting model should be biased towards reducing wastage. On the other hand, for an ambient product such as canned food in a high-footfall store, the selection of the forecasting model should be biased towards reducing out-of-stock instances.
Typically such optimizations are achieved with frequent manual interventions over time, but they are prone to errors and often complete neglect. With AI, however, model selection can be done automatically based on best fit in line with your category objectives.
Forecasting with advanced techniques such as ML and deep learning, and ensemble-based approach of combining multiple models, has proven to be much more accurate than traditional forecasting frameworks.
Integrating with Customer Data Systems and Curating an Attractive Assortment
With the ever-evolving nature of customer data systems that capture a variety of data—from social media, eCommerce sites, apps, POS, etc.—it has become imperative to integrate this information in your demand planning, so your shelves appear attractive and fresh to customers every time they visit.
With traditional forecasting frameworks, it is nearly impossible to integrate this information into your demand planning due to the sheer complexity of the depth and width of categories.
But with digital technologies such as AI/ML, all this information can be fed seamlessly into your demand planning system. The outcome – your customers get a delightful shopping experience customized as per their preferences.
Key Advantages of AI-powered Demand Forecasting
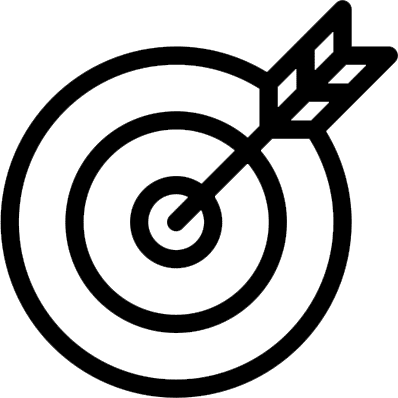
Improvements in Data Forecasting Accuracy
Over time, Machine Learning algorithms learn from historical data trends, and with more data being fed to the system, the data forecasts become better. Any new emerging pattern in demand is automatically fed into the ML engine, thereby making it more robust and adaptive.
Moreover, the AI-assisted features help planners with data treatment, feature engineering, and selection. As a result, 90% of categories show instant improvement in accuracy as compared to traditional time series-based forecasting.
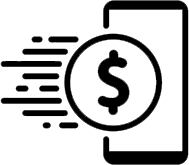
Effective Replenishment
With accurate SKU-level forecasts and retail-tuned replenishment optimization solutions such as Algonomy’s Order Right, retailers have been able to reduce out-of-stock instances by 75%, wastage by 30%, and inventory cost by 10%.
Order Right optimizes order plans for your supply chain constraints such as lead time, minimum order quantity, and category factors such as expiration date, etc.
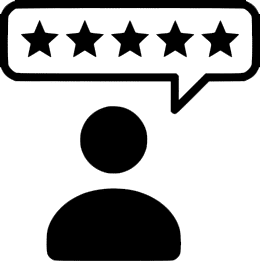
Increased Customer Satisfaction
Nothing is more frustrating for a customer than out-of-stock and expired products on the shelf. Demand-driven forecasting and supply chain-optimized replenishment lead to improved shelf availability.
In addition, demand-sensing capabilities help category managers catch on to emerging trends before competitors and eliminate non-performing SKUs, making sure that shelves appeal to customers.
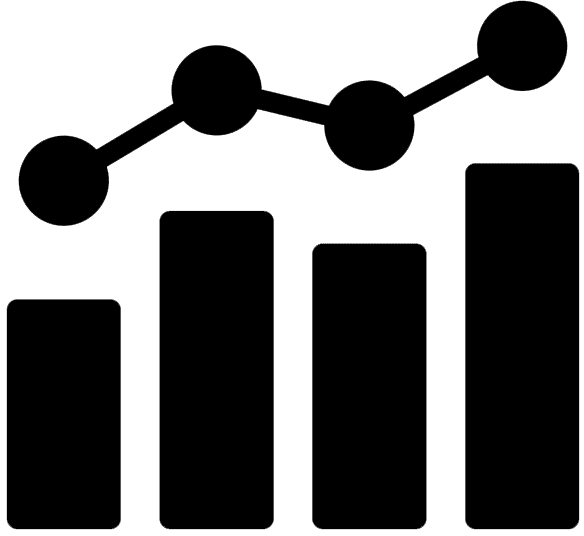
Improved Workforce Productivity
Your category managers show improved productivity when they spend more time on strategic aspects of improving margins and achieving growth rather than working on difficult-to-use dashboards and tools.
Using an AI-based demand forecasting solution that is business-user friendly has proven to make the entire forecasting process up to 100x faster.
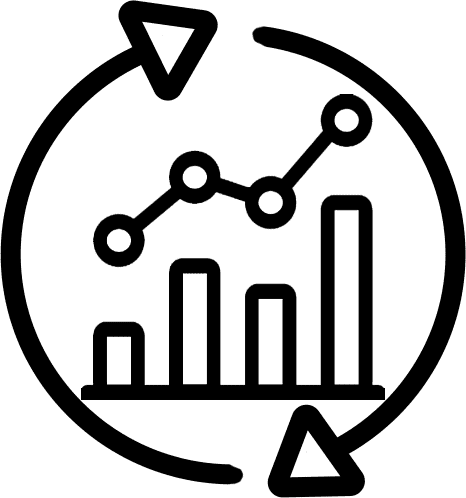
Effective Markdown/Discount Optimization
Retailers often incur higher inventory holding costs when products remain unsold for longer periods. There’s a risk of products hitting their expiry date and losing value. Ultimately, the retailers have to sell these products at a discounted price leading to margin erosion.
This scenario is greatly minimized with an AI-based forecasting framework which not only reduces forecasting errors but also builds demand sensing capabilities which allows category managers to react to scenarios playing out on the shelf in a swift fashion.
Key Considerations for Choosing the Right Demand Forecasting Solution
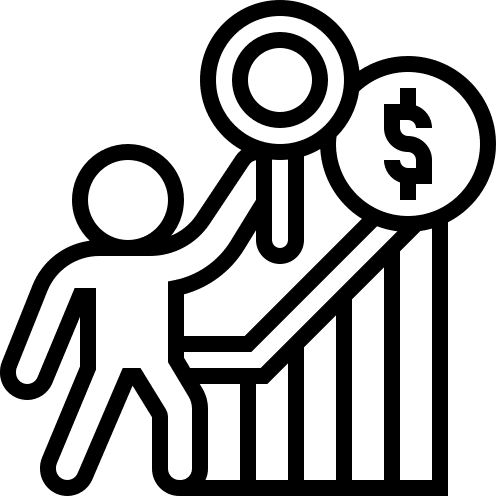
Demand Indicators
The solution must account for the full spectrum of demand forces such as historical trends, external factors such as weather conditions, macroeconomic factors, etc., and internal factors such as promotions, pricing, etc., without tedious configurations that require frequent tech support.
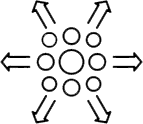
Retail-tuned ML Algorithms
Since each store, category, and product is different, the solution must use an ensemble of algorithms with a built-in feature engineering and selection engine. The focus should be on achieving category objectives rather than simply chasing accuracy.
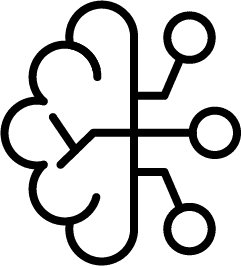
AI-powered Automation
To reduce dependence on manual intervention and technical understanding, the solution should use AI-based automation to reduce the burden on category managers and simplify the decision-making process.
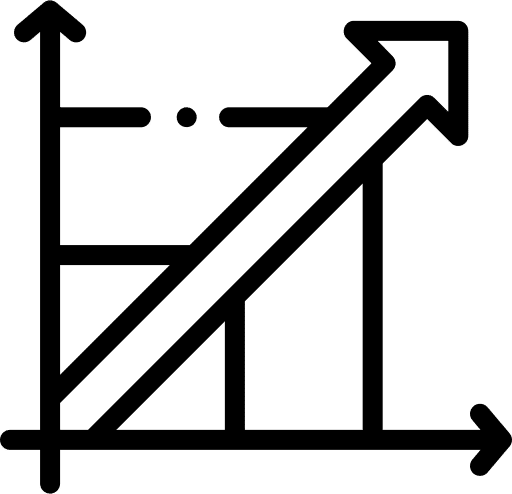
Scalability
A retail solution is fed large sets of data daily. As data continues to evolve in terms of volume, variety, and velocity, the complexity is here to stay. Therefore, your demand forecasting solution must be scalable to ingest and transform data and use AI/ML and other advanced analytics to provide actionable insights.
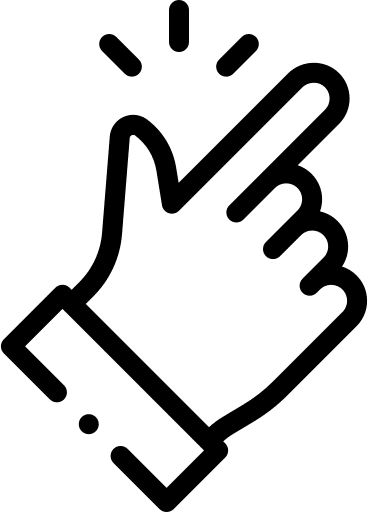
Easy to Use
Category managers and supply chain planners might not necessarily be tech-savvy. However, they should be able to instantly use advanced features to forecast demand and react to grocery situations without depending on data scientists and analysts.
If the demand planners require extensive training on the demand forecasting solution to deliver value, they will revert to unproductive behaviors and your returns on the technological investments will be extremely low.
Retail Demand Forecasting With Algonomy - How Can We Help?
Algonomy is the only AI and ML-powered platform for algorithmic decision-making across all retail verticals – merchandising, marketing, and digital, and offers a wide range of solutions for different use cases.
Algonomy’s Forecast Right leverages ML-based multivariate and algorithmic techniques to accurately and adaptively forecast future demand in grocery retail. Its advanced AI capabilities offer feature engineering and model selection for demand forecasting, with a proven track record of improving forecast accuracy for over 90% of SKUs.
Retailers can take their replenishment planning to the next level by plugging the results from Forecast Right into another excellent offering – Order Right, a highly intelligent AI-powered solution to generate accurate SKU-level order plans.
Order Right considers key supply chain factors such as lead time, minimum order quantity, etc., and category factors such as shelf-life, expiration date, etc., while constantly monitoring stock balance, sales, and demand predictions, to create highly granular and accurate insights for strategic planning.
With Algonomy’s powerful solutions, retailers can break the vicious cycle of hunch-based planning and static data modeling and unlock:
- 90% forecast accuracy at a granular level
- 100X faster forecasting capabilities
- Tailor-made feature engineering and model selection for retail
To know more about our products, or to catch them in action, please get in touch with our team f experts and schedule a free personalized demo session today!