Download this article as a PDF
Grocery retail supply chains are getting more complex and unmanageable with traditional forecasting models. Matching supply with demand for a broad inventory that includes fresh and short shelf-life products on one end and ambient products on the other is not easy. Add to that the complexity that arises due to changing consumer behavior, who have started to incline towards convenience and price over brand loyalty. Yet traditional demand forecasting is still heavily reliant on constant monitoring and intervention from a supply chain expert.
Accurate and agile demand forecasting lies at the center of grocery retail’s customer-centric yet lean approach. Doing forecasting right has far-reaching benefits:
- You reduce your wastage by better inventory demand planning
- Your displays look attractive and dynamic
- Customers get fresher goods
- You sell more by placing your product at the right place at the right time across channels
So, let’s look at nine secrets to improve your demand forecasting and take it to the next level.
1. Account for Dynamic Demand Forces With Multivariate Forecasting
With price sensitivity and convenience changing the way consumers shop, the demand for products has become much more volatile and difficult to predict with simple models. It has therefore become imperative for grocers to enrich data and not simply rely on traditional data. For accurate forecasting, it’s crucial to account for external factors such as weather, holidays, events, social media, and news as well as internal factors such as promotions, advertising, visual merchandising, etc.
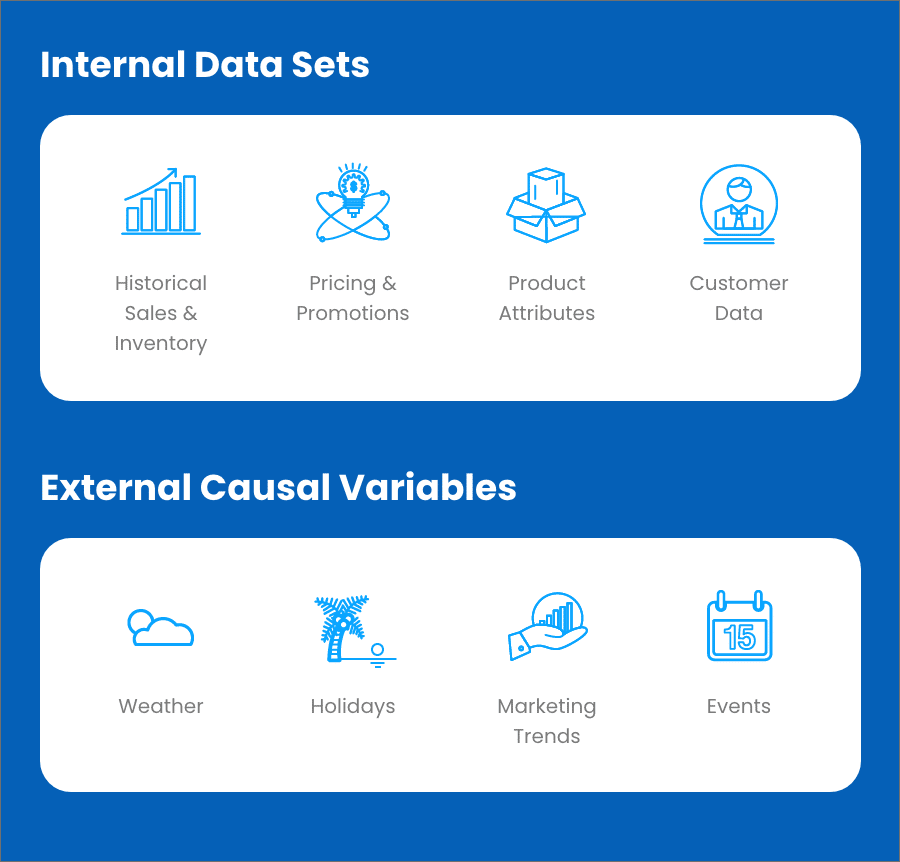
2. Let ML Do the Heavy-lifting and Help You Decide What Factors are the Most Important
With a huge range of internal and external causal variables affecting sales, every store, channel, and category combination behaves differently. One of the biggest mistakes that grocers make is to force-fit models without understanding the nuances that are at play.
In a multivariate framework, it is very difficult and cumbersome to determine the importance of each factor manually. ML algorithms, however, can help to sift through data and determine the effect of each factor. This can then feed in as an input for the planner to generate granular and accurate forecasts.
3. Go the Extra Mile on Forecast Accuracy With an Ensemble of Algorithms
While forecasting sales of products, there is a slim chance that you will find a silver bullet algorithm that works for all products, locations, and situations. Therefore, champion grocers go the extra mile with an ensemble of algorithms that is customized based on the data. This ensures that grocers avoid over-fitting of models across product lines and achieve greater overall accuracy.
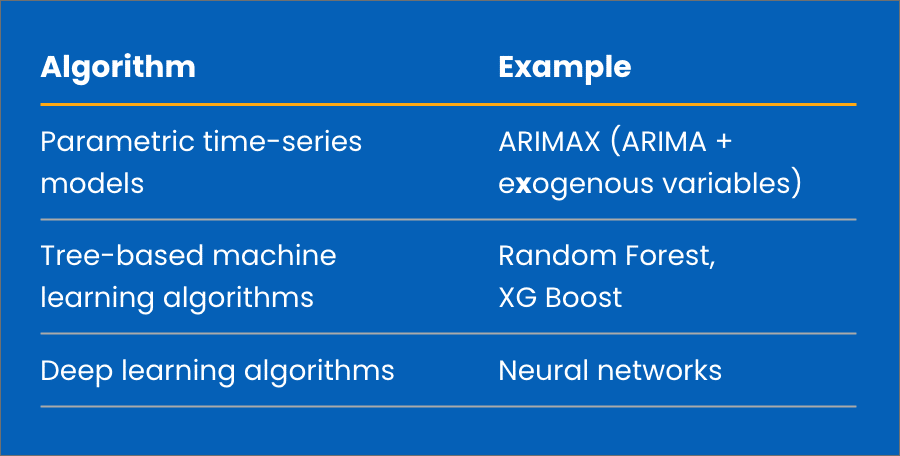
4. Adopt a Dynamic Approach to Fresh and Ambient Products With Business Objectives as Priority
From fresh goods wholesalers to grocery retailers, from high-end to price-driven supermarkets, convenience stores to cash-and-carry chains, it is clear that replenishment teams walk a tightrope between spoilage costs and shelf presentation. This makes it important to get the balance right every time.
Error functions such as RMSE and MAD are powerful tools that can be used to select the best model by analyzing the prediction error. Such methods are indifferent to over-forecasting and under-forecasting. However, depending on where the product lies in the fresh to ambient spectrum, these functions can be tuned to treat over-forecasting and under-forecasting differently based on the business requirement and impact.
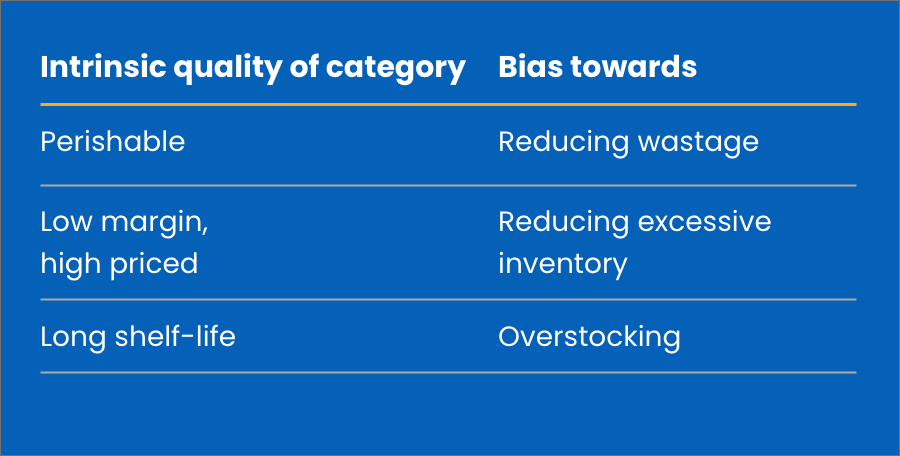
5. Tie Your Forecasting to Outcomes
What should be your forecasting accuracy? Should it be above 95%? Or 99%? The correct answer to this question is not so simple.
Most forecasting techniques aim to achieve the highest accuracy levels, giving very low importance to business outcomes. Tying your forecasting to outcomes such as reducing wastage, overstocking, or increasing availability has helped several leading retailers achieve great success even with forecasting accuracy as low as 70%.
6. Pro-actively Adapt to In-store Scenarios
While managing stocks at grocery stores, it is critically important for store managers to respond to what is happening on the shelf. For instance, a new product launch could lead to secondary effects on the demand for other products, which could range from an overstock situation in case of cannibalization to understock in case of multi-buy discounts. For true agile operations, business users should be able to swiftly identify and plan for such situations on a daily basis without the need of technical support.
Events that cause a secondary effect on other products:
- Multi-buy discounts
- Price change
- Promotions
- Advertising
- Change in in-store display
- Product launches and discontinuation
- Macro-level scenarios such as weather, local events
7. Don’t Discount the Cannibalization Effect
The effect of promotions of products via price discounts, advertisements, display changes, etc. on the supply chain is one of the least studied topics but has huge implications. For example, the promotion of one product may have significant effects on the sales of other products that are not in promotion. Not accounting for this effect leads to suboptimal supply chain planning and ill effects like increase in spoilage or overstock. Promotion forecasts can go a long way to satisfy the increase in demand while mitigating the ill effects.
8. Sparse and Noisy Data Is the Norm, Not an Exception
With increased new product launches, fresh products, and increasingly complex channels, sparse and noisy data is a recurring theme across grocers worldwide. If you regularly face the roadblock of not having enough quality data for your planning needs, then it is time to look for a solution. Invest in a forecasting framework that uses data science techniques to deal with sparse and noisy data with ease.
9. Scalability Is Not Optional Anymore
Irrespective of you taking a top-down or a bottom-up approach to your demand planning, you will eventually have millions of demand forecasts at the SKU-store level. This planning is getting even more unmanageable due to changing consumer behavior and channel factors. Therefore, top grocers realize the importance of making sure that the system is scale-ready, both from a technical and user experience point of view.
In the ever-evolving, dynamic, and volatile grocery retail, your demand sensing and forecasting framework needs to be intelligent, agile, and scalable to be able to deal with the above-mentioned challenges. One such solution is Algonomy’s Forecast Right.
Forecast Right uses proprietary ML-based multivariate and algorithmic techniques to accurately and adaptively forecast demand. It is 100X faster and scalable than traditional forecasting solutions – 5-clicks is all it takes to generate 1000s of granular forecasts. Its proprietary AI provides tailor made feature engineering and model selection for demand forecasting and has a track record of improving forecast accuracy for over 90% of SKUs.
The output of a solution like Forecast Right can be plugged into various use cases. One such use case is replenishment planning. Powered by Forecast Right, Algonomy’s Order Right generates accurate SKU-level order plans for even the most challenging categories – from fresh and seasonal to new and promoted products with ease. It does so by leveraging proprietary optimization algorithms that constantly monitor stock balance, sales and demand predictions while accounting for constraints such as shelf-life, lead-time, expiration date, minimum order quantity, minimum display stock, and standard ordering frequency constraints.
Also read: How Machine Learning Improves Retail Demand
Learn more about Algonomy’s Forecast Right and Order Right.