Accurate inventory forecasting is the heart of retail planning and inventory optimization. Capturing demand accurately and optimizing inventory accordingly can not only reduce inventory costs but can also help retailers boost customer satisfaction, improve shelf availability, and increase inventory ROI.
With fierce competition, rapidly evolving customer preferences, and quick new product and brand introductions, retailers can no longer afford to plan inventory manually.
Studies reveal that the annual costs of holding excess inventory can reach up to 32% and recent economic changes, evolving priorities, etc., are pushing as many as 75% of customers to change their shopping habits.
So, how can retailers forecast demand, capture demand patterns, and evaluate the effect of seasonal variations on the inventory? What is inventory forecasting and what are the different techniques for the same? How important is it to forecast inventory and what are some of the best practices that retailers can benefit from?
Below, we explore the answers to all these questions.
What Is Inventory Forecasting?
Inventory forecasting is predicting the required inventory levels for a future period based on historical sales data, customer purchase trends, upcoming events, etc. Forecasting involves drawing well-calculated projections based on data-driven insights from previous data and multiple constraints influencing customer purchase behavior and demand.
Often used interchangeably with demand forecasting inventory forecasting spans beyond setting reorder points and order plan adjustments based on seasonal sales influencers. It encompasses identifying patterns and trends to adapt to dynamically evolving customer demands and market conditions to curate the right inventory at the right time to meet customer demand optimally.
Inventory Forecasting vs Inventory Replenishment vs Demand Forecasting
While all three terms are interconnected and generally used interchangeably in the retail ecosystem, they are distinct processes
Inventory Forecasting
Inventory Replenishment
Demand Forecasting
Inventory Forecasting
- Predicting future inventory requirements based on historical sales data, seasonal variations, and market trends
- The goal is to estimate optimal stock levels for meeting anticipated customer demand.
- Effective strategies include tracking and monitoring inventory KPIs – reorder points, average inventory, etc.
Inventory Replenishment
- Predicting future inventory requirements based on historical sales data, seasonal variations, and market trends
- The goal is to estimate optimal stock levels for meeting anticipated customer demand.
- Effective strategies include tracking and monitoring inventory KPIs – reorder points, average inventory, etc.
Demand Forecasting
- Predicting future inventory requirements based on historical sales data, seasonal variations, and market trends
- The goal is to estimate optimal stock levels for meeting anticipated customer demand.
- Effective strategies include tracking and monitoring inventory KPIs – reorder points, average inventory, etc.
Why Is Inventory Forecasting Important?
Inventory forecasting helps retailers plan and optimize inventory and cater to customer demand such that overstocks, stockouts, and wastage are reduced, and shelf availability is increased.
Forecasting inventory prepares retailers with reliable insights to adapt their replenishment plans and inventory distribution according to near real-time factors and sales influencers. They can club the insights with demand forecasting insights and replenishment plans to minimize the impact of supply-side deviations on inventory and sales.
1. Reduce Inventory Costs
Traditionally demand planners and inventory managers use static planning methods which lead to overstocks or OOS events, both of which cost retailers their business. While overstocking eats away at the working capital, it also balloons up warehouse storage costs and leads to obsolescence and complete wastage in certain categories.
Likewise, OOS events affect customer experience and impact the revenue pipeline. Studies reveal that as many as 73% of customers will change retail brands after just one bad experience and a whopping 70-90% of stockouts are caused by poor shelf replenishment practices.
With data-driven inventory prediction retailers can minimize stock issues, order and allocate optimal inventory levels across all geographies, and reduce inventory costs. AI-driven inventory replenishment solutions with built-in forecasting engines can take the inventory optimization process one step further by offering highly granular forecasts for specific product-location entities.
2. Overcome Data Inaccuracies
As many as 40% of retailers cancel at least one out of ten orders due to inaccurate inventory data. Overcoming retail data inaccuracies is particularly challenging owing to the highly disparate functioning of all system functions. Further, the retail data is infamous for being highly unstructured, noisy, and riddled with gaps.
Overcoming data inaccuracies is essential for accurate inventory forecasting because any errors in capturing inventory or demand data have a snowball effect on the final inventory values and metrics.
Intelligent retail demand planning and forecasting solutions inherently overcome such challenges by employing the intelligence of advanced data analytics techniques. They run on intelligent algorithms that are fine-tuned to the nuances of retail data and can generate inventory plans with high forecasting accuracy by eliminating noise and gaps.
3. Improve Inventory ROI
McKinsey reveals that AI-driven forecasting can reduce supply chain errors by 30 to 50%, shrinking lost sales by 65% and warehousing costs by 10 to 40%. All these are crucial factors affecting the overall inventory ROI and can lead to significant cost savings for retailers.
Further, PwC outlines that investing in solutions that help optimize data-driven use cases, such as customer data, can increase their contribution margins by 3% to 5% after deducting initial investments and acquisition costs.
What makes inventory forecasting so beneficial is the fact that it accounts for the interplay of multiple factors and constraints, ranging from sales patterns to customer purchase patterns, demand shifts, demand lifts, seasonality, external/internal factors, and more, to generate optimized inventory plans.
So, ultimately, the retailers are able to boost revenue, sales, customer satisfaction, and shelf availability, while reducing stock issues, wastage, and inventory.
Inventory Forecasting Techniques
There are multiple methods of inventory forecasting. Further, as retailers use different forecasting solutions with different capabilities, the boundaries among the techniques, algorithms, and processes are fleeting and evolving with every passing day.
Generally, inventory forecasting techniques can be categorized into the following four categories:
Trend Forecasting
- Shows trend projections based on changes in product demand over time
- Doesn’t always account for seasonality or sales data irregularities
Graphical Forecasting
- Identifies patterns and sloped trend lines to find out insights that might have been possibly missed
- Unable to handle complexities, heavily subjective, and reliant on historical data
Quantitative Forecasting
- Uses statistical methods and historical sales data to generate future inventory projections
- Demonstrates data dependency and inability to adapt to change
Qualitative Forecasting
- Involves market research and focus groups to predict future inventory requirements
- Doesn’t always account for seasonality or sales data irregularities
Different Techniques for Inventory Forecasting
1. Time-Series Forecasting
This inventory forecasting method involves predicting future demand patterns based on historical data, and past inventory levels. It works well for categories with consistent and measurable inventory patterns.
The process involves data collection, data processing, trend analysis, checking the effects of seasonality on data, and forecasting model selection. It is important to note that the model selection is going to have a significant impact on the overall accuracy and efficiency of forecasting and is done based on specific characteristics of inventory data.
Common techniques for time series inventory forecasting are moving averages, Seasonal Decomposition of Time Series (STL), exponential smoothing, deep learning approaches, etc.
2. Seasonal Forecasting
This is one of the most popular inventory forecasting techniques and it predicts future demand patterns in inventory data showing a recurring seasonal effect. For instance, trending categories or products during holiday seasons, seasonal cosmetic products, agricultural products, fashion apparel, etc.
Its ability to identify seasonal trends and variations in demand enables retailers to plan for seasonal fluctuations and adjust inventory levels accordingly. Coupled with accurate demand forecasting and replenishment techniques, it can help retailers reduce inventory holding costs, stockouts, and overstocks, and boost customer satisfaction with the right inventory at the right time.
Common techniques for seasonal inventory forecasting methods include Historical Averages, and Machine Learning and Deep Learning-based approaches.
3. Historical Data Analysis
A stepping stone for demand forecasting, the historical data analysis technique involves exhaustive analysis of historical data, be it sales, inventory, market, etc., and offers valuable insights into customer behavior, top-performing categories, sales patterns, and many more critical business metrics.
However, historical data analysis is a challenging process as the data sets tend to become highly unstructured and full of noise and gaps over time. Hence, the technique requires data cleaning, data organization, and standardization, etc., to improve the data quality.
Unless coupled with advanced data analysis and processing techniques, the technique can lead to erroneous projections due to the data cleansing and standardization steps.
Common techniques for historical data analysis include regression analysis, cyclical patterns, time series analysis, exponential smoothing, trend analysis, etc.
Inventory Forecasting Techniques Based on Statistical Models

Time Series Modeling
- ARIMA (Autoregressive Integrated Moving Average) Model
- SARIMA (Seasonal ARIMA) Model
- Exponential Smoothing Model
01
02
Inventory Forecasting Techniques Based on Statistical Models
Regression Modeling
- Logistic Regression Model
- Multiple Regression Model
- Linear Regression Model
- Polynomial Regression Model
- Ridge and Lasso Regression
- Bayesian Regression
Challenges in Choosing the Right Inventory Forecasting Techniques
Data Quality and Preprocessing
As mentioned above, the retail data is noisy, has gaps, and comes with multiple redundancies. This means, that pre-processing or standardizing data for quality purposes can affect the accuracy of results. This is mainly due to the fact that the models can reject the correct or relevant data or consider noise as the correct data.
As every next step in the forecasting process is built on top of the results drawn from data cleansing, the effects can easily snowball into massive errors, derailing the accuracy of inventory forecasts and ultimately business decisions.
How to Handle Sparse and Noisy Data in Retail Forecasting
Seasonality and Trends
Vetting the effect of seasonality or trends on specific data can be particularly challenging owing to subjective data handling and analysis. When done incorrectly, the model can over-fit or underfit data patterns affecting the overall forecast accuracy.
On the other hand, seasonality might be relevant for a specific geography or set of consumers as well. For instance, the seasonal variations in the demand for challenging products like skincare are extremely complex to monitor and predict.
Model Selection and Tuning
While statistical modeling is one of the best inventory forecasting methods, model selection and data tuning can become subjective, thereby causing distortion in actual results. Manual model selection and tuning to business-specific use cases is a tedious process, which can spur business risks affecting revenue and profitability.
Dynamic Demand Patterns
Demand Planning for the modern retail ecosystem requires an understanding of the complex interplay of multiple factors, such as historical sales, in-store promotions, economy, trends, micro-trends, and supply-side disruptions. Add to the mix, the location and category-specific sales influencers and constraints for optimization, and the retailers are left with nothing short of a Pandora’s Box.
Further, the demand patterns change dynamically due to multiple external and internal factors, such as the emergence of omnichannel platforms, quick delivery services, and hybrid operation categories, which, can steer the forecast accuracy in the wrong direction
Leverage AI-Driven Retail Planning to Manage Promotional Chaos
Other miscellaneous factors such as data scarcity, multi-seasonal patterns, short product lifecycles, tough categories like ultra-fresh, fast new product introduction, etc., make inventory forecasting particularly challenging and vulnerable to errors.
How to Approach Inventory Forecasting – Best Practices
As evident from the above discussion, there is no single best inventory forecasting technique, as demand patterns are highly dynamic in nature, keep on evolving, and are affected by multiple factors.
Further, traditional and manual forecasting suffers from inefficiencies, inaccuracies, and poor scalability. Coupled with additional factors, like lead times, fill rates, and demand deviations stemming from in-store promotional dynamics, retailers find it difficult to generate precise inventory plans.
This is where AI and ML-driven inventory optimization comes into the picture.
The AI/ML-driven inventory optimization solutions come with built-in intelligence of thousands of algorithms that can control and optimize for multiple factors and constraints, enabling retailers to unlock unparalleled precision and control over inventory.
Why Choose Optimization Instead of Standalone Inventory Management or Demand Forecasting?
Inventory optimization involves optimizing inventory at granular levels, precisely at the product-location level for multiple categories, stores, SKUs, and so on. What makes inventory optimization superior to standalone inventory forecasting and demand forecasting techniques is the fact that retailers can unlock immaculate precision and control over inventory based on accurate predictive modeling and real-time adjustments via a single interface.
Algonomy’s Order Right is one such powerful inventory optimization platform that comes with a built-in demand forecasting engine and ensemble AI algorithms to predict demand based on hundreds of customizable parameters.
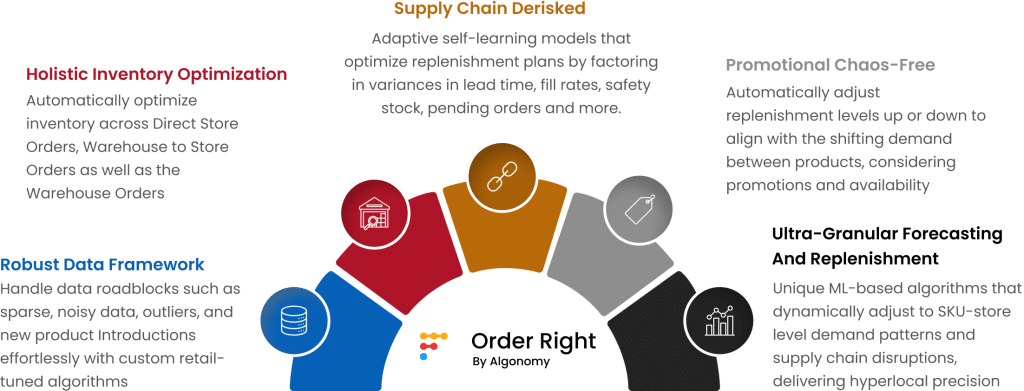
Robust Data Framework
Handle data roadblocks such as sparse, noisy data, outliers, and new product Introductions effortlessly with custom retail-tuned algorithms
Holistic Inventory Optimization
Automatically optimize inventory across Direct Store Orders, Warehouse to Store Orders as well as the Warehouse Orders
Supply Chain Derisked
Adaptive self-learning models that optimize replenishment plans by factoring in variances in lead time, fill rates, safety stock, pending orders and more.
Promotional Chaos-free
Automatically adjust replenishment levels up or down to align with the shifting demand between products, considering promotions and availability
Ultra-granular Forecasting and Replenishment
Unique ML-based algorithms that dynamically adjust to SKU-store level demand patterns and supply chain disruptions, delivering hyperlocal precision
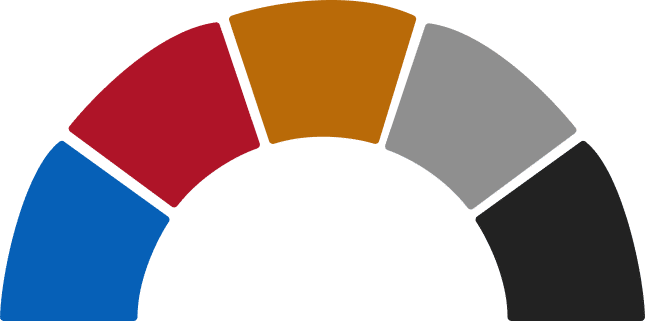
Order Right (OR) enables retailers to generate accurate SKU-level order plans with unique retail-tuned replenishment algorithms for intelligent demand-based replenishment planning.
Powered by Forecast Right which uses proprietary ML-based multivariate and algorithmic techniques to accurately and adaptively forecast demand, Order Right generates accurate SKU-level order plans for even the most challenging categories – from fresh and seasonal to new and promoted products with ease to help businesses unlock:
- 10% Reduction in Inventory Cost
- 75% Reduction in Out-of-Stock Instances
- 10-30% Reduction in Wastage
- 99% Increase in Shelf Availability