Lessons in eCommerce search from a major online retailer
If you’re as picky as I am, you probably spend a lot of time researching several products to ensure you make the best decision. But when was the last time an eCommerce store showed pertinent information that helped you make a purchase decision?
This happened to me some time ago when I was on an online store to find a pair of noise-canceling headphones. I used the search bar and was pleasantly surprised to see a headphones comparison article pop up, in addition to relevant headphones. Thanks to the timely and relevant article that showed up, I was able to speed up this process.
Most businesses think of eCommerce personalization only in terms of product recommendations. However, it’s equally important to personalize other key touchpoints in the online shopping journey — search, browse, and content. Doing so allows for a more holistic customer experience, which translates to increased conversions and revenue per visit. eCommerce search is increasingly becoming a key pillar of CX.
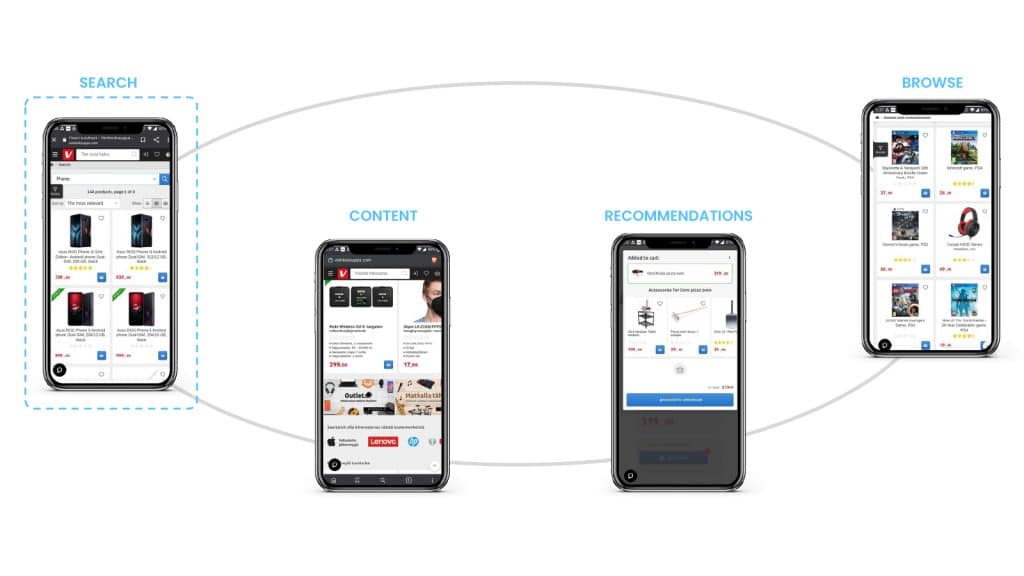
Why? For one, shoppers who search convert 3x more than those who don’t. This has to do with the fact that people who use search have an express intent of what they want to buy.
Personalization should kick in as a shopper types their search query — showing products that they are more likely to be interested in based on their individual behavior and known preferences. But before getting to personalized results, the basics need to be in place, and this requires effort and is not without its challenges.
Let’s look at some common challenges with traditional search engines through the eyes of one of our clients: Verkkokauppa.com. Verkkokauppa.com is Finland’s most-visited online retail store with 65,000 SKUs in 26 product categories.
Challenges with Traditional Search Engines
1. Inability to Handle Product Data Issues
Traditional search engines primarily rely on product metadata and descriptions to display results.
It is quite rare for a shopper to make precise, descriptive search queries. For example, a person might search for ‘computer’, which is an umbrella term that could refer to a PC or a laptop. Even ‘laptop’ is a rather broad search term, as there are various categories like Notebook, Ultrabook, Convertible, and Netbook. In such cases, the search engine needs to work with limited information and decide what to show the shopper.
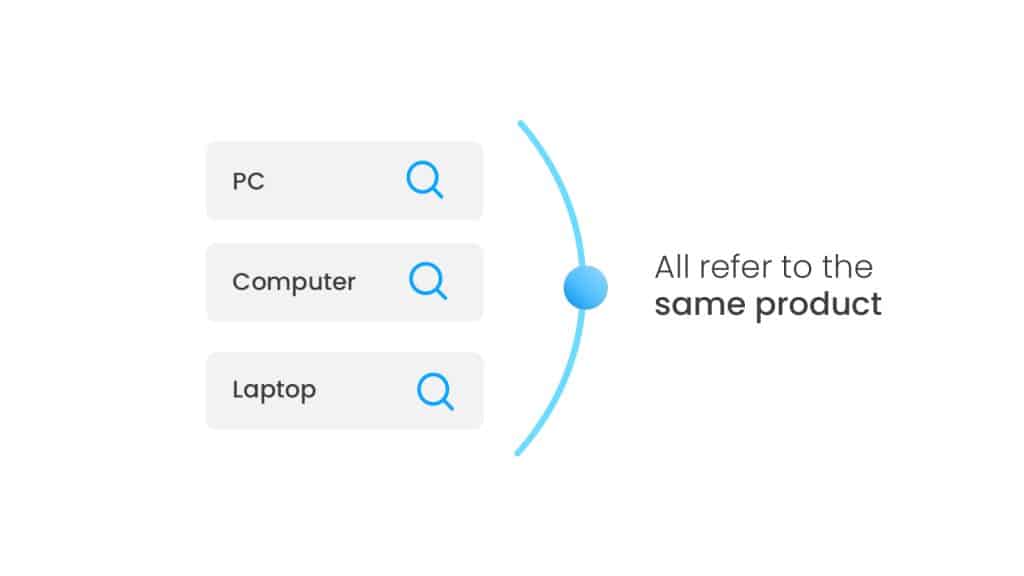
To overcome this, developers need to create synonyms to match products to specific keywords. However, this is a very time-consuming approach, and sustaining it just isn’t practical.
2. Irrelevant Search Results
Our client also recalled an experience when he searched for ‘iPad’ because he was looking for, well, an iPad. But the initial results showed products like iPad sleeves and other accessories — items he didn’t need. He had to scroll further down to find the actual product. For a traditional search engine, these results are technically correct because their product metadata contains the word ‘iPad’, but the results are not relevant to the shopper’s current need.
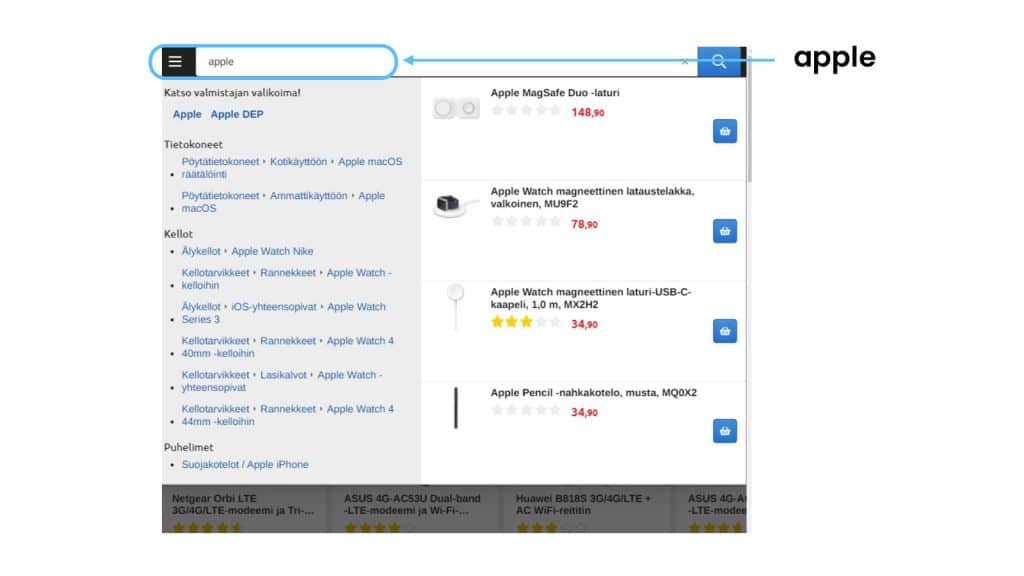
3. The No-results Page
Another common issue is shoppers mis-typing the product or model name, leading to bad search results. But what’s worse is the dreaded ‘No results’ page. When a shopper sees this page after a search query, they have basically hit a dead end. This is bad for business. It’s important to always show relevant alternatives even if the shopper’s desired product isn’t available.
Download Personalized Search Case Study
Época Cosmeticos Pivots to Individualized Shopper Experiences, Improves SEO with Personalized Search, Content and Recommendations.
Download Now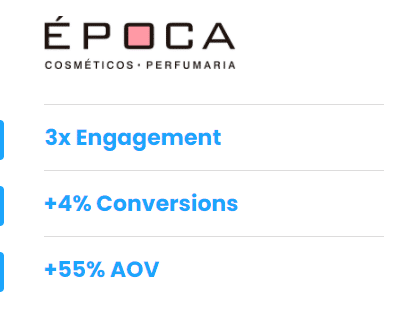
How Verkkokauppa.com Managed Product Data Issues and Improved Search Relevance
Let’s see how Verkkokauppa addressed the aforesaid data issues with self-learning, personalized search.
Anton Paasi, Head of eCommerce at Verkkokauppa.com, discusses the potential of eCommerce Search at Algonomy Personalization Summit 2021
Wisdom of the Crowd
When a shopper searches for ‘Apple’, the results could display every Apple product available. But is that relevant to the shopper? Perhaps not. One way of addressing this is to look at the collective behavior of shoppers after they searched for ‘Apple’ and tune the results accordingly. This is the essence of what’s known as Wisdom of the Crowd (WoC).
WoC uses a Machine Learning algorithm to learn from the entire shopper population, their queries, and what they view or purchase subsequently. And the results are, more often than not, accurate.
In a recent conversation, Verkkokauppa.com shared how leveraging WoC helped them bring relevance to the results when the search queries were as short as one word.
Take the example of the word ‘lamppu’, which is Finnish for a light bulb. The issue with the word ‘lamppu’ is that it’s also used as a compound word for several categories of lamps — such as ‘taskulamppu’ which means a torch, and ‘älylamppu’ which refers to a smart lamp.
Figure 4 below shows the search results for ‘lamppu’ before the application of WoC. The results show various types or categories of lamps because the product titles contain the word ‘lamppu’. While the results are technically correct, they aren’t relevant.
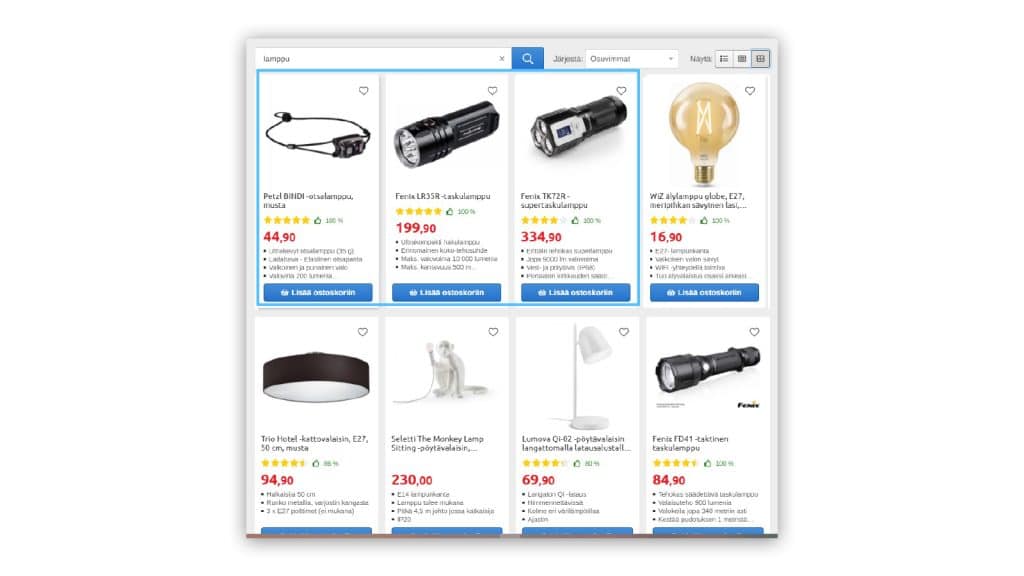
Figure 5 shows WoC in action. The search engine now knows that people using the search query ‘lamppu’ are in fact looking for a light bulb. So it displays several options for a light bulb at the top of the results and pushes the other categories of lamps below.
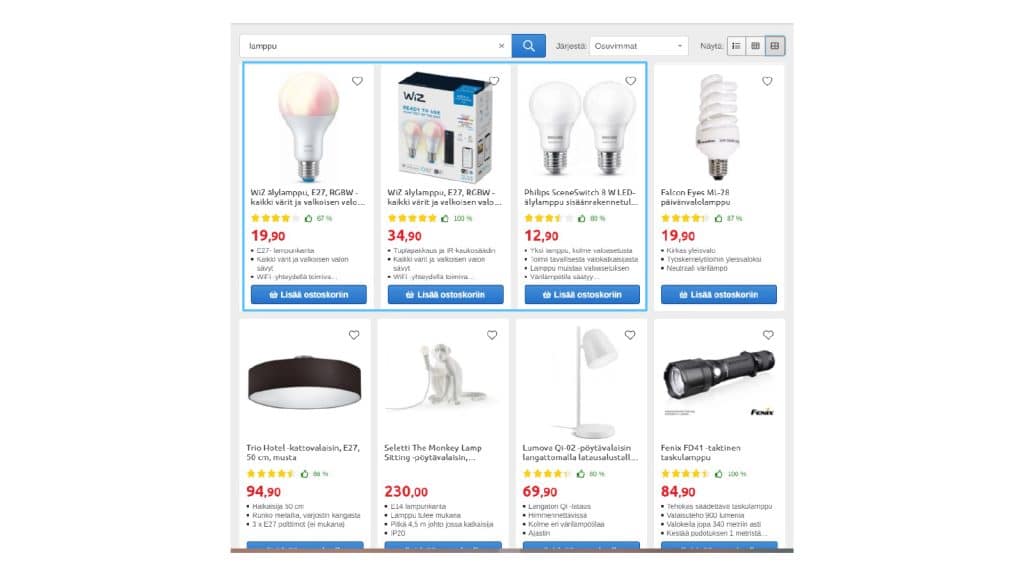
The WoC functionality, therefore, helps understand shopper terminology like a human would, and boosts relevant items, while eliminating the dependency on developers to build various rules.
Personalizing Search Results with Behavioral Data
Leveraging behavioral data to drive 1:1 personalization is now table stakes. Search personalization helps convert shoppers with clear purchase intent quicker.
Verkkokauppa experienced a 10-15% increase in conversions when they made the switch from one-size-fits-all search to personalized search.
The retailer uses Algonomy Find™, an eCommerce personalization software that not only helps deliver richer personalization but also improves product discovery, with the use of behavioral data. It leverages behavior attributes (see Fig. 6) to organize search results, as per the individual’s intent, in real time.
The personalization software allows for granular controls that help set weights and experiment with each of these attributes. This is as simple as moving sliders. The software, therefore, empowers business users to adjust personalization settings themselves as and when needed without any dependency on IT.
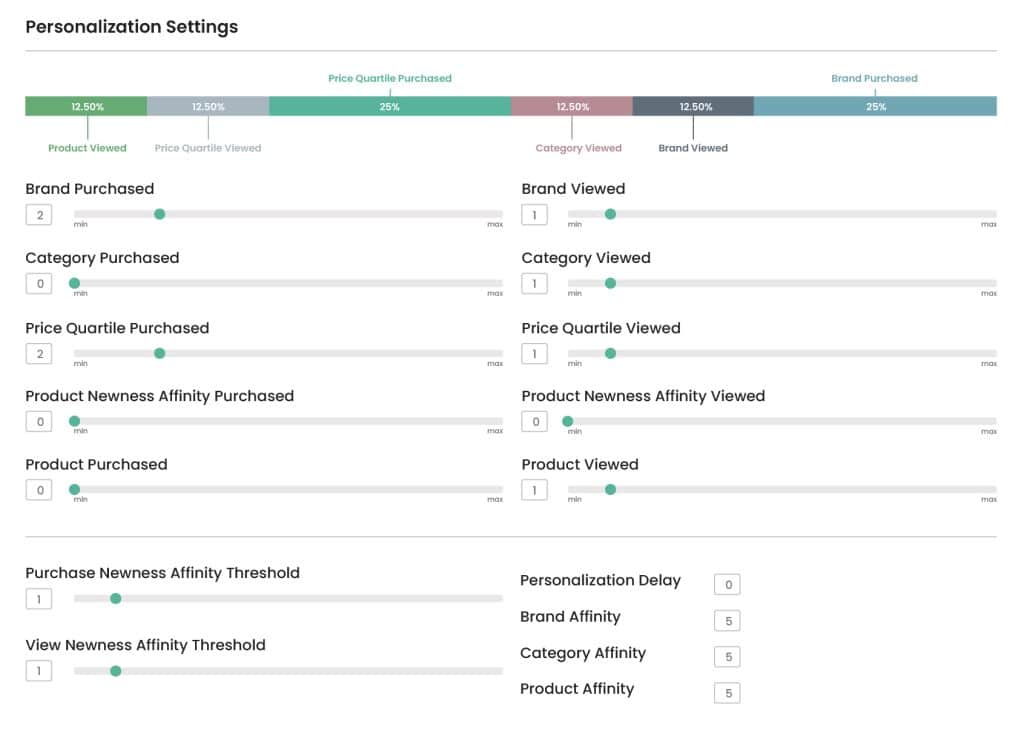
In a world where consumers interact with digital commerce even when the actual purchase is made in-store, it’s more important than ever for retailers and brands to invest in customer experience strategically, and across the entire shopper journey.
While devising an eCommerce personalization strategy, retailers must think beyond product recommendations and recognize that search is no more just a developer tool, but a lever for business growth.