Why Read the Whitepaper on Trend-based Guard-Railing in Demand Forecasting?
Every year, grocery retailers lose $1 trillion due to stock unavailability and $750 billion in wastage. This demand-supply mismatch is primarily due to huge forecasting errors caused by changing customer expectations and channel complexities.
Machine Learning-based forecasting methodology captures data beyond the historical sales data to include other key factors such as weather, events, and macroeconomic factors, which improves accuracy. ML models have proven to be more accurate than traditional demand forecasting models in over 90% of categories.
However, under-forecasting and over-forecasting are inevitable in certain product categories. That’s when guard-railing comes in handy. Guard-railing is the process of setting up boundaries such as not forecasting below zero and not forecasting above a maximum value.
In this whitepaper, we talk about how setting up a trend-based guard-rail mechanism can help improve accuracy for up to 25% of demand forecasts. We also talk about how this mechanism can be implemented.
What You Will Learn from the Whitepaper
- How machine learning-based forecasting is more accurate than traditional models.
- What trend-based guard-railing in demand forecasting is.
- How to implement a trend-based guard-railing mechanism.
- How the right forecasting solution can help you achieve this easily and quickly.
Download the whitepaper to learn more about how Algonomy Order Right can make your forecasts more accurate, agile, and adaptive.
Read the Whitepaper
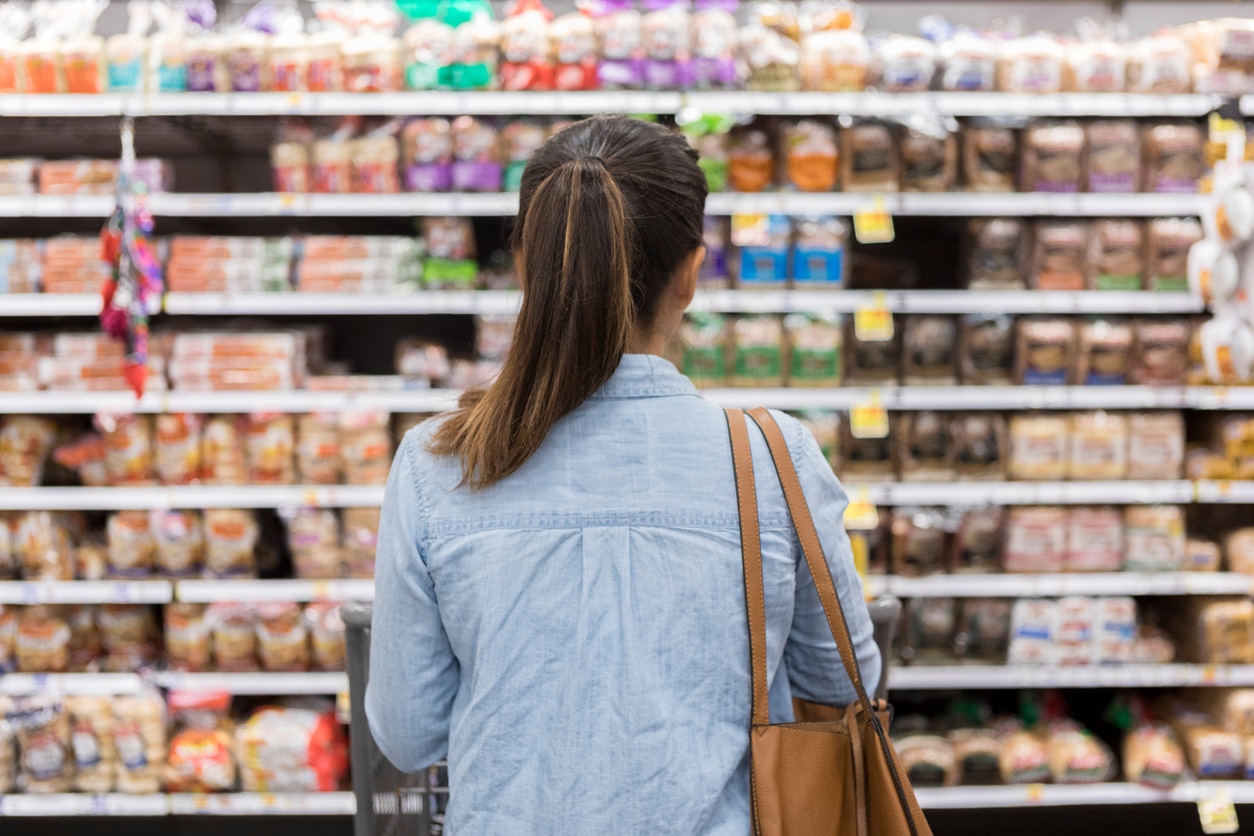