Segment
Fashion and Apparel
Challenge
Reducing inventory spending and preventing loss of sales due to sub-optimal assortment mix
Product Used
Outcomes

range planning
efforts

overstock
and understock

variance between range and financial plan (from 12%)

full price
sell-through

end-of-season
leftovers
Overview The client is one of the leading fast-fashion retailers with 700+ stores across the US. The client offers apparel, accessories, and footwear targeting young men and women looking for affordable and trendy fashion. The client faced challenges with its existing assortment planning processes:

Assortment range and size planning required 840,000 man-hours every month for 300+ product groups across stores.

The large deviation between range plan and financial plan resulted in overstock of 1.6 million units.

Ad-hoc merchandise size planning led to frequent out-of-stock instances and customer dissatisfaction.
The client was looking for an easy-to-use, intelligence-driven, and proven algorithmic solution to optimize assortment and achieve merchandising financial goals.
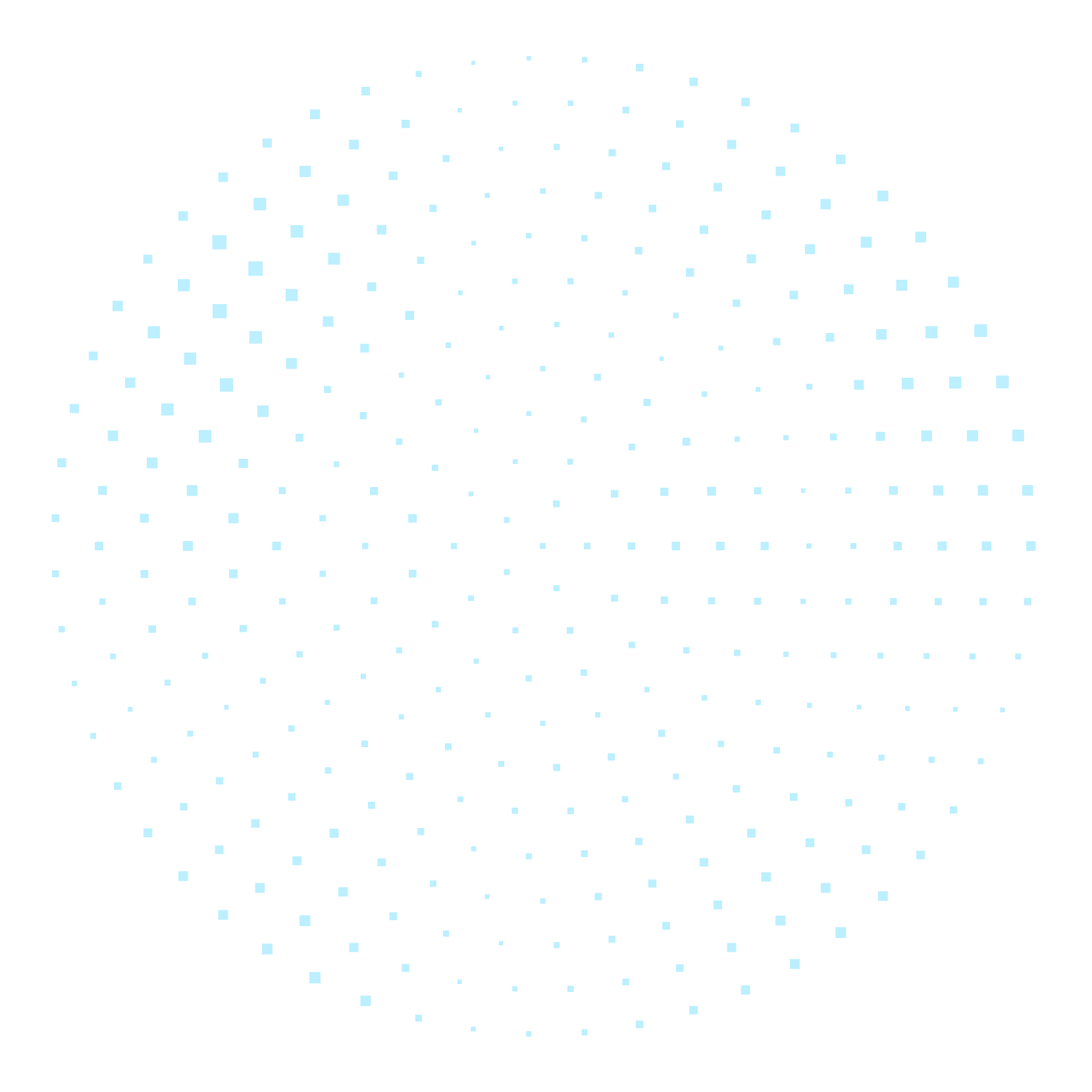
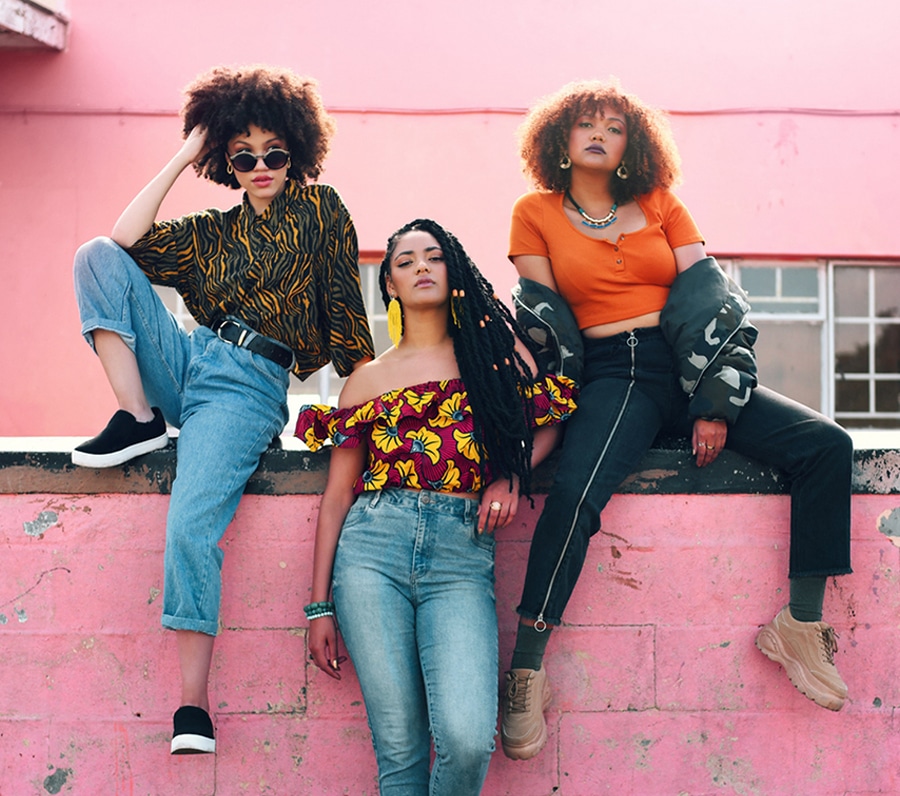
Algorithmic Decisioning Platform for Assortment Planning
Algonomy Assortment Edge (AE) was the perfect fit for the client’s requirement. AE is designed to expedite and optimize the process of building an optimized assortment. With its smart 1-click automation, AE helped the client automate the time-consuming process of planning and the advanced AI algorithms recommended a demand-driven assortment breadth, depth, and size pack – minimizing markdowns, controlling inventory spendings, and delighting customers.
Our ML-based ensemble of algorithms provided highly accurate, granular, and attribute-based sales forecasts. Additionally, it performed store clustering based on key dimensions such as product class, attributes, consumer segment, etc.
The ROI of Algorithmic Decisioning
The client made the transition from an ad-hoc manual assortment planning to a demand-driven assortment planning, powered by intelligent features of Algonomy Assortment Edge:

With the help of smart automation, the range planning for each store was reduced to a click from the earlier 4 hours, resulting in savings of over 840,000 man-hours per month.

Algorithmic range plan recommendations helped achieve the ideal assortment breadth and depth at store level, reducing the variance between financial and range plan from 12% to 3%.

With size pack recommendations by store, cluster, and region, the client optimized the size curve and introduced multiple size packs suited to meet the demand, reduce overstock, and understock by 4%.
With AE’s smart automated workflows and ML-based algorithmic assortment optimization, the client was able to improve the productivity of its assortment planners by 700%, achieve 3% higher full-price sell-thru, and reduce end-of-the-season leftovers by 2%.
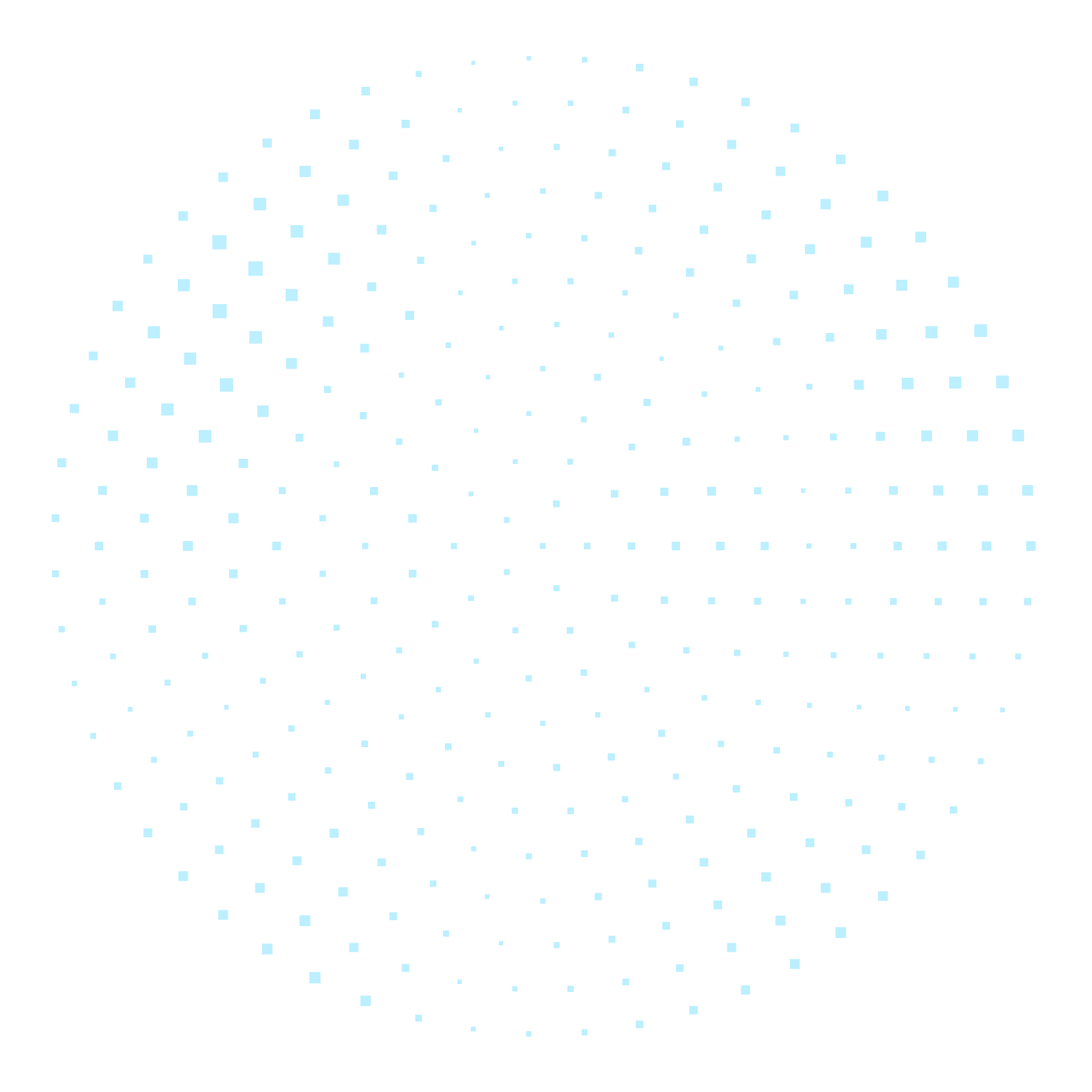
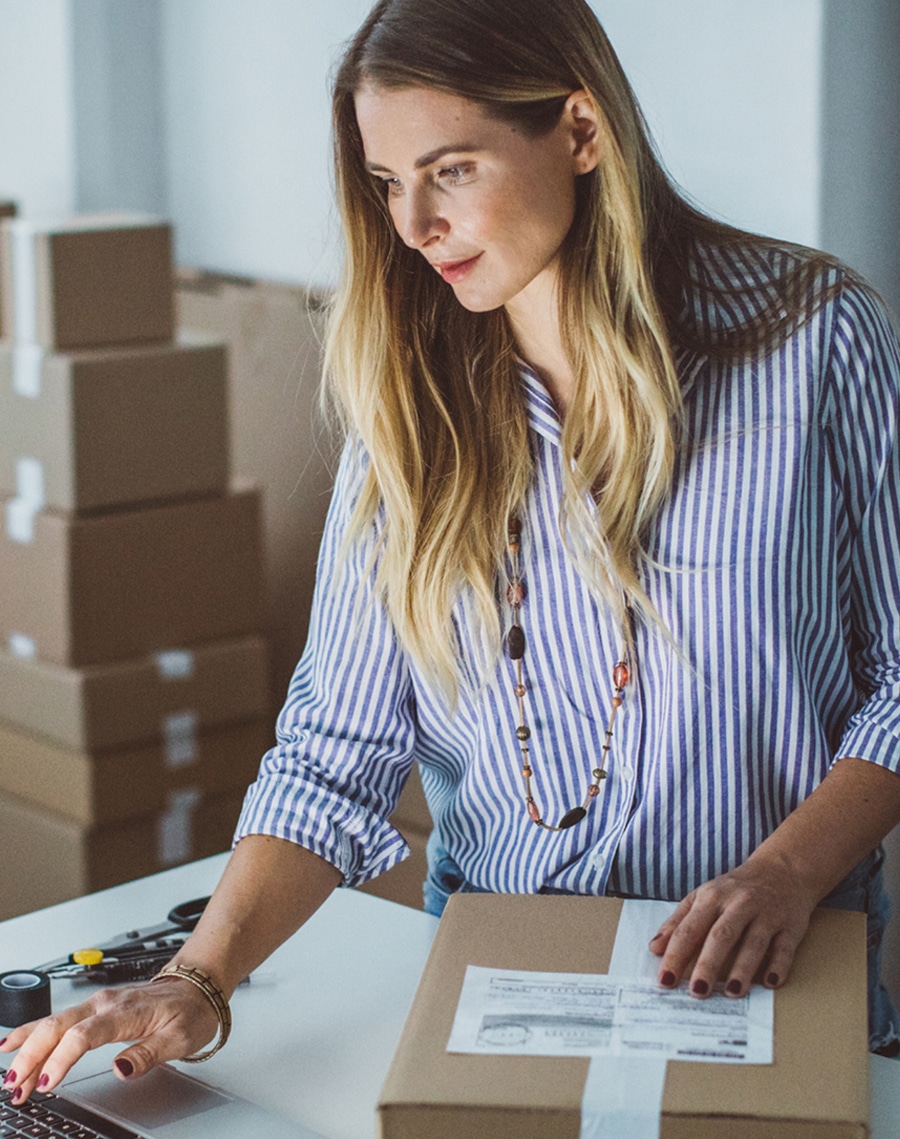
Need Help With Your Assortment Planning? Let's Talk.
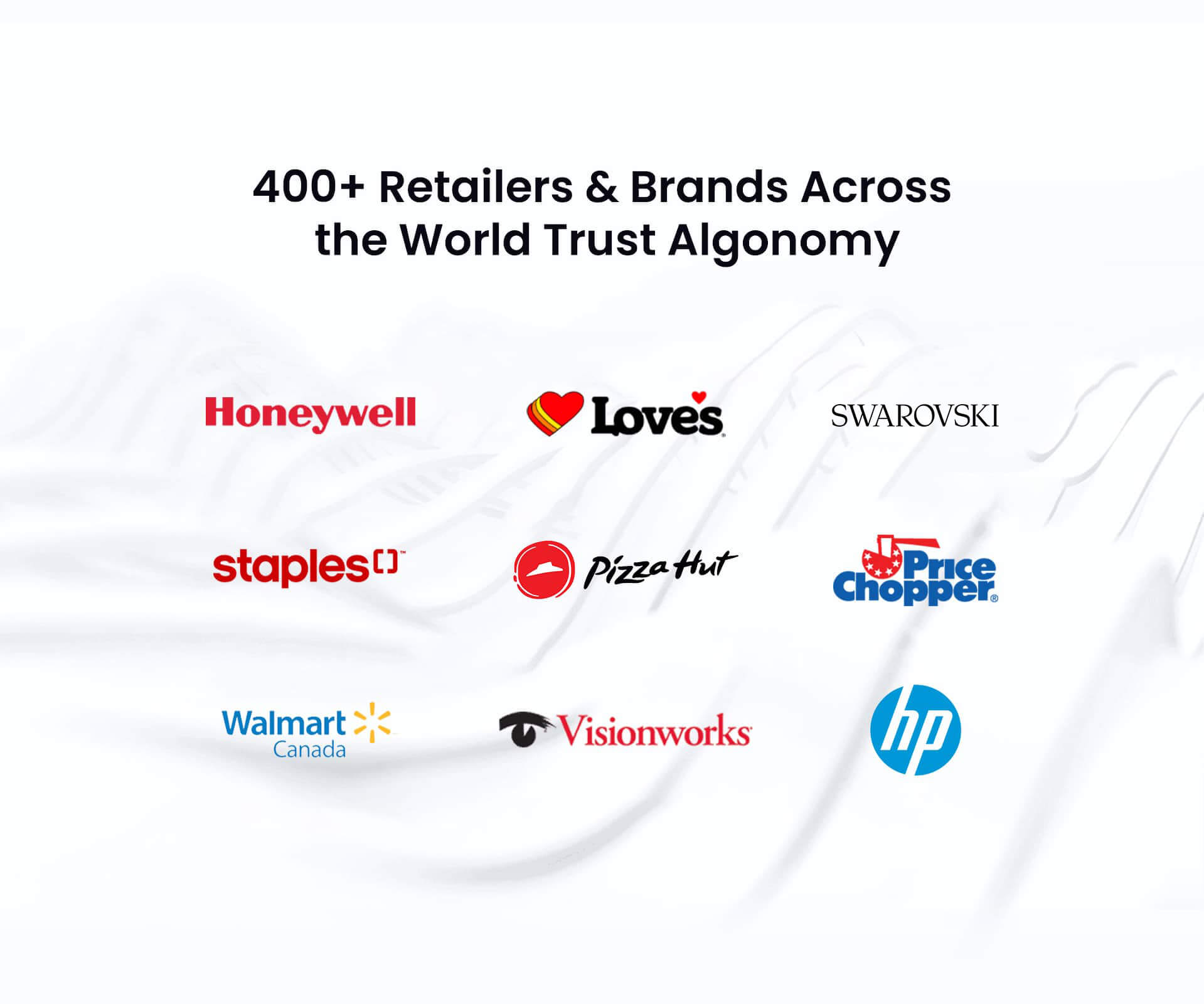